Case Study
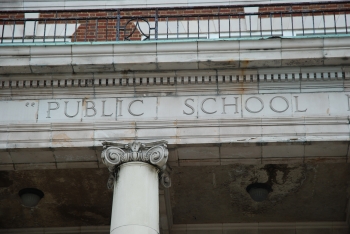
Challenges
Educational policy at the national, state, school district and even school levels depends on the large education data bases that are publicly available, especially those of the National Center for Education Statistics (NCES). Making sure that interpretations of these data are accurate and that the methodology used to report and to draw inferences do so correctly is essential to a solid basis for setting educational policy and standards. Making sure that appropriate methodology is available to the users of these data is critical.
A research collaboration amongst statisticians, psychometricians, education researchers tackled key areas related to analysis of NCES data in general and test results from NAEP (National Assessment of Education Progress) as a specific case. They analyzed the strengths and weaknesses of general methods often used for education data; and then they focused on development of new, correct statistical methods for analyzing complex surveys in the field of education. Problems considered included: How to compare in a fair way NAEP results for school districts with differing populations and widely different demographics? How many times can the same data be used for analysis of different objectives? How to improve upon a simple statistic like an overall mean when some population subgroups are disproportionately represented or are under-represented in the data? How to distinguish simultaneous occurrence from causality in large education data sets? How do analytic methods differ when seeking to test (in order to either confirm or reject) a hypothesis and when exploring to generate new conjectures (hypotheses)?
Answering these questions and providing new methodology for correctly making inferences gave education data analysts new tools for answering basic questions facing education policy makers.
Research Project
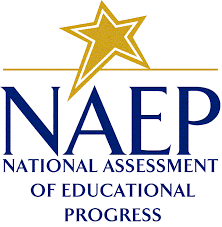
NISS was hired by the NAEP to develop new statistical methods to analyze complex surveys in the field of education. NISS developed more effective designs for answering basic questions facing education policy. A group of researchers including statisticians, psychometricians, and education researchers examined existing data sets and focused on issues related to national educational performance and its connections to demographic characteristics of students. They explored general issues in simultaneous and casual interpretation.
Workshop(s): Inferential Issues in Large Educational Data Sets, October 1996
Paper(s):
Williams, Valerie, Kathleen Billeaud, Lori A. Davis, David Thissen, and Eleanor E. Sanford. "Projecting to the NAEP Scale: Results from the North Carolina End-of-Grade Testing Program." Journal of Educational Measurement 35, no. 4 (1998): 277-296. Abstract.
Shaffer, Juliet Popper. "Multiple Hypothesis Testing: A Review." Annual Review of Psychology 46 (1995): 561-584.
de Leeuw, Jan, and Ita G. G. Kreft. "Questioning Multilevel Models." Journal of Educational and Behavioral Statistics 20, no. 2 (1995): 171-189. Abstract.
Williams, Valerie S. L., Lyle V. Jones, and John W. Tukey. "Controlling error in multiple comparisons, with special attention to the national assessment of educational progress." Journal of Educational and Behavioral Statistics 24 (1999): 42-69. Abstract.
Technical Report(s):
Technical Report 23: Multiple Hypothesis Testing: A Review
Technical Report 31: Questioning Multilevel Models
Technical Report 33: Controlling Error in Multiple Comparisons, with Special Attention to the National Assessment of Educational Progress
Technical Report 34: Projecting to the NAEP Scale: Results from the North Carolina End-of-Grade Testing Program
Technical Report 35: Perspectives on Statistics for Educational Research: Proceedings of a Workshop
Technical Report 65: Comparing Regression Coefficients Between Models: Concepts and Illustrative Examples
Principal Investigator(s): Jerome Sacks, Ingram Olkin, Daniel Horvitz, Lyle Jones
Senior Investigator(s): Stephen Rauderbush, Leon Gleser, Larry Hedges, Eugene Johnson, Eva Petkova
Post Doctoral Fellow(s): Valerie Williams