Research Project
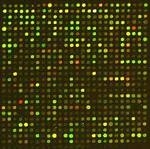
Emerging OMICS technologies such as microarray and highthroughput screening have been adopted across a broad spectrum of applications as these technologies are capable of simultaneous measurements of very large numbers of channels. Thus they enable rapid experimental turnaround with an accompanying promise to extract the small number of relevant elements or factors from a massively larger number of inert ones. In doing so, they also pose many challenges for reliable and reproducible statistical analysis and scientific interpretation: high dimensionality with n<<p, sparsity, computational challenges, and an excess of false positives. These difficulties pervade two distinct challenges: to analyze these data in order to isolate active elements and to model predictively from one or more large data bases.
The first illustrative project uses microarrays to identify and validate sex-specific genetic biomarkers, in this case, for gonad development. Gene expression data at various key stages of fetal mouse testis development have been collected, but finding the significantly up-regulated (or down-regulated) gene expressions during this development requires sophisticated statistical analytic tools. This is complicated because genetic biomarkers for critical gonadal developmental activities are expected to be specific to each stage of development while other unrelated gene activation may be either random or related to other co-synchronous fetal developments.
Predictive modeling tackles a different problem - in the case studied here, it requires data mining capability across combined but unrelated large, sparse data bases. An enormous number of environment chemicals remain untested for safety due to constraints on financial and biological resources. To address the issue of prioritizing chemicals for evaluation of toxic potential and/or carcinogenicity, the United States Environmental Protection Agency opened the ToxCast program. The goal is to design a twostep testing program that uses high-throughput in vitro screening to assess responses across multiple assays in order to select the chemicals to evaluate with conventional in vivo testing. The immediate challenge is to construct predictive models using the high-throughput in vitro features in the ToxCast Phase I dataset coupled with information from extensive chemical data bases on chemical properties that may be relevant to predicting the in vivo toxicity of chemicals not included in the ToxCast data.
Analyze microarray data to find significant genes as input to pathway analysis. Build predictive models of toxicity using high-throughput in vitro bioassay data.
NISS assembled bioinformatics expertise, statistical applications of matrix theory, and software experience with omics and computational chemistry analysis algorithms group to join scientists working in genetics and biochemistry to build multidisciplinary research teams: Stan Young (NISS), Jessie Xia (NISS postdoc), Kevin Gaido (Hamner), Rusty Thomas (Hamner).