Research Project
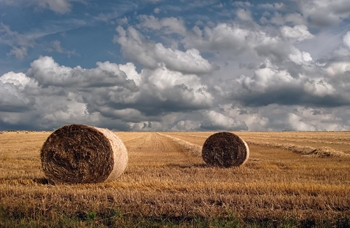
The National Institute of Statistical Sciences (NISS) established a Cross-Sector Research in Residence Program in partnership with the National Agricultural Statistics Service (NASS), the survey and estimation arm of the U.S. Department of Agriculture. This new collaborative venture by NISS and the USDA was the first project of a NISS initiative to host academic-government research teams focused on specific federal agency objectives.
Each team of five people comprised of a faculty researcher in statistics, a NASS researcher, a NISS mentor, a postdoctoral fellow and a graduate student who worked intensively together at NISS during the summers of 2009 and 2010 to solve research questions posed by NASS.
Varied projects focused on advances in statistical methodology for implementation in USDA surveys and analysis of survey results.
Multivariate Imputation Mechanisms and Valid Mean Squared Error Estimation: Agricultural Resource Management Survey – Phase III
One of the objectives of the Agricultural Resource Management Survey – Phase III was to allow statisticians and economists to conduct multivariate statistical analyses of the farm economy with valid estimates for the potential error in model estimates and forecasts. NASS has been using a univariate approach to both imputation and mean-squared-error estimates, but multivariate approaches were needed to support multiple estimates and simultaneous forecasts for multiple crops. This team worked on developing a multiple-imputation scheme that could handle the complexities associated with heterogeneous data, and also the semi-continuous nature of agricultural data. The second challenge was to determine the validity of the method when the prediction models underlying its imputation fail.
New Design and Estimation Methodologies for Biased Self-Exclusion (Under-coverage): Estimation of Small Farms from Census Mail List
NASS accounts for the incompleteness of its Census Mail List (CML) by adjusting the weights of Census respondents to capture the estimated number of farms identified on the area-frame, but not on the CML. When the 2007 Census was processed, NASS also identified several valid farms that were not found in the area-frame, even though they were located in sampled area segments. This poses the question of how many farms are missed by both sources, CML and area-frame. The challenge was to develop statistical procedures to measure the number of farms missing from both frames and to incorporate these into Census weights. Cognitive issues were also addressed since many qualifying small farms do not necessarily consider themselves farms and hence fail to return the survey forms.
New Statistical Editing and Imputation Methods That Preserve Data Quality: Quarterly Agricultural Survey
NASS utilizes data cleaning/editing procedures in many of its surveys that are based on an expert opinion/analysis review process and manual intervention to correct identified data values outside of normally expected ranges. This manual editing process is time consuming and is not consistent. It can lead to edit effects that are not reflected in the measurement error process. The objective for this project was to create automated statistical/selective editing and imputation strategies that could reduce the non sampling errors and lower the survey cost by reducing the extensive staff resources currently used in the data cleaning process.
Statistical Multi-Source Predictive Models and Error Estimates: Major USDA Crop Protection Forecasts and Estimates
The USDA produces multiple forecasts of crop protection throughout the growing season and estimates production at the end-of-season or after harvest. Information is collected from multiple sources (USDA surveys and administrative/auxiliary information, including weather and remotely sensed data) and then synthesized by a panel of experts in USDA’s Agricultural Statistics Board (ASB) resulting in the official forecasts/estimates that are published. These forecasts are compared to the utilization of the crops and assessed for accuracy. Subsequently, when the actual yields are known, can improvements be made to this process via increased use of data modeling or through other approaches? How can these models or other techniques be validated during the short time period analysts have to review the inputs and publish the time sensitive official estimates?
The research teams examined these various focus areas over two consecutive summer periods. The program started in the summer of 2009 when the complete teams met at NISS.The postdoctoral fellow and graduate student spent the summer at NISS working on the project under NISS mentorship, with periodic meetings with the faculty member and the NASS researcher. During the academic year, the postdoctoral fellow resided at the USDA, continuing the work with the NASS researcher. In the summer of 2010, the teams met again at NISS and completed their work.
Postdoctoral Fellows: Patricia Gunning, Michael Robbins and Jianqiang Wang
Help the National Agricultural Statistics Service come up with more efficient ways to better count farms across the United States.
Team One:
Sujit K. Ghosh, North Carolina State University,
Barry Goodwin, North Carolina State University,
Darcy Mille, NASS,
Tim Keller, NASS,
Peter Quan, NASS,
Kirk White, USDA Economic Research Service,
Michael Robbins, NISS, and
Joshua Harbinger, NISS.
Team Two:
Linda Young, University of Florida,
Pam Arroway, North Carolina State University,
Andrea Lamas, NASS,
Denise Abreu, NASS,
Patricia Gunning, NISS, and
Kenneth Lopiano, NISS.
Team Three:
Balgobin Nandram, Worcester Polytechnic Institute,
Scott Holan, University of Missouri,
Wendy Barboza, NASS,
Edwin Anderson, NASS,
Jianqiang (Jay) Wang, NISS, and
Criselda Toto, NISS.