Research Project
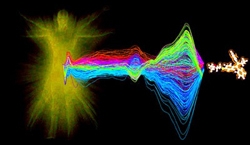
NISS has joined the Clinical Proteomic Technologies for Cancer research initiative that focuses on technologies for cancer biomarker discovery. The overall goal is to foster the building of an integrated foundation of proteomic technologies, data, reagents and reference materials, and analysis systems. This will systematically advance the application of protein science to accelerate discovery and research in cancer. Progress in cancer proteomic research has continually been hampered by a lack of standardized technologies and methodologies which are critically needed for effective discovery and validation of proteins and peptides as biomarkers. The challenges to the Clinical Proteomic Technology Assessment for Cancer (CPTAC), a collaborative network of proteomic centers plus the National Institute of Standards and Technology, are to (1) conduct a rigorous assessment of two major technologies for protein and peptide analysis – mass spectrometry (both shotgun and MRM) and affinity capture platforms; and (2) develop and validate technical tools for general use in proteomics that ensure reliable and reproducible results, analyses and interpretations.
A statistical tool box has been developed for repeatability and reproducibility analysis for the data obtained from LC-MS/MS experiments. Furthermore, statistical models have been constructed to detect the system sources of instability and to adjust the bias in the data obtained. The ultimate objective is to employ appropriate and efficient statistical approaches to improved diagnostics, therapies and even prevention of cancer.
An automated algorithm has also been developed to detect systematic instabilities in instrument and liquid chromatography performance during MRM experiments. This algorithm was used to assess peptide retention times, a key parameter in MRM
studies, especially those employing scheduling LC-MRM-MS. Four new system stability metrics have been constructed to detect outliers, drift and shift from a sequence of replicate sample injections with multiple peptides. The algorithm is intended to be used to evaluate the instrument and liquid chromatography performance before and during the experiment. It has now been further extended to dynamically predict the retention time window based on previous system status and retention times. This allows setting up the retention time window between batches of runs.
While the requirement for a normalization step during label-free analysis of “shotgun” proteomics data sets had previously been established, no study to understand the nature of the systematic biases affecting peptide intensities has been undertaken. A semi-parametric statistical method has been developed as part of this project to rank the effects of system and peptide property variables in order of importance and provide a robust method to normalize the data. Understanding the nature of peptide intensity biases and improving methods to process label-free data can help establish these methods as robust solutions for biomarker discovery in complex biological mixtures.
Beside measurement of peptide intensities, spectral counting is an alternative measure used in label-free quantitative proteomics.“Shotgun” studies are designed to identify peptides present in a biological sample, typically using spectral counts from a LCMS system. Spectral counting is used in other applications as well, but there are several challenges inherent to LC-MS spectral counting in biomarker discovery; i.e., few replicates, sparse counts, a large number of proteins, and unreliable variance estimation. A Bayesian hierarchical model with mixture priors is used to model and analyze these data. Compared to other methods for spectral count analysis in the literature, the new approach provides higher sensitivity for a given false positive level.
CPTAC Institutions
Broad Institute of MIT and Harvard
Memorial Sloan-Kettering Cancer Center
Purdue
UC-San Francisco/Lawrence Berkeley National Laboratory and Buck Institute
Vanderbilt School of Medicine
Publications and Invited Presentations (Refereed)
[1] Integrating LC-MS/MS data across instrument platforms for a common sample. D. L. Tabb, X. Wang, L. J. Vega-Montoto, P. A. Rudnick, M. C. Chambers, C. R. Kinsinger, H. Rodriguez, D. C. Liebler, N. Sedransk, S. E. Stein. (In review for submission).
[2] Monitoring System Stability in MRM Proteomics Study. X. Wang, L. Zimmerman, X. Feng, N. Sedransk (submitted).
[3] Systematic Biases Affecting Peptide Intensities in Label-free Shotgun Proteomics. P. Rudnick, X. Wang, X. Yan, [others], N. Sedransk, S. Stein, and CPTAC (in review for submission).
[4] Bayesian Models in Biomarker Discovery Using Spectral Count Data in the Label-Free Shotgun Proteomics.X. Wang and N. Sedransk (submitted).
Develop and build statistical models to solve a sequence of critical questions in shotgun and multiple reaction monitoring (MRM) proteomics: (1) evaluate repeatability and reproducibility of liquid chromatography–mass spectrometry (LC-MS) experiments; (2) diagnose the system instabilities; (3) adjust the systematic bias in peptide intensities; (4) detect biomarkers.
NISS senior Directors, experienced in biostatistics, “omics” computational technology, metrology andcalibration: Nell Sedransk and Stan Young. NISS postdoctoral fellows with specific expertise in bioinformatics, statistical computation, and statistical applications for “omics” and other large scale data: Xingdong Feng, Jessie Xia, and Xia Wang