Research Project
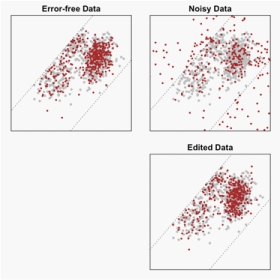
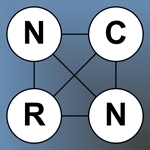
The Triangle Census Research Network (TCRN), established by NISS and Duke University, develops broadly-applicable methodologies intended to transform and improve data dissemination practice in the federal statistical system. It focuses primarily on methods for (1) handling missing data and correcting values in large complex surveys, (2) disseminating public use data with high quality and acceptable disclosure risks, and (3) combining information from multiple data sources, including record linkage techniques.
Edit-Imputation of Microdata
Publicly released microdata by federal agencies potentially support complex and rich secondary analyses that lead to deeper scientific understanding and more informed policy making; yet, data quality often suffers because data subjects are reluctant to respond to surveys or are prone to report data with errors. Statistical agencies frequently handle nonresponse with ad hoc approaches that tend not to work well in complex datasets with many variables—especially when data are released to the public—because they often ignore multivariate relationships. Similarly, editing faulty data is frequently based on heuristics rather than principled theory.
NISS and Duke researchers have developed a general framework for simultaneous imputation of missing data and editing of faulty data.. This framework adopts nonparametric Bayesian methodology to reflect complex distributional features of collected data. By integrating paradigms from statistics and operations research, the resulting edit-imputed data are guaranteed to satisfy logical constraints provided by domain-experts. The approach is applied to data from the Census of Manufactures, where we demonstrate the improvement of our procedures over existing approaches to edit-imputation.
Data Confidentiality
Most federal agencies view disseminating data to the public for secondary analyses as a core mission; yet, concerns over data confidentiality make it increasingly difficult to do so. As threats to data confidentiality grow, federal agencies planning to produce public use data may be forced to release heavily redacted files. Many confidentiality protection strategies applied at high intensities result in severely reduced data quality. Even worse, analysts of secondary data have no way to determine how much their analysis has been compromised by the disclosure protection.
NISS and Duke researchers have extended the theory and methodology for releasing multiply imputed, synthetic datasets based on flexible, nonparametric Bayesian models. We generate synthetic data that preserve features of the joint distribution while respecting linear constraints among variables. Ongoing research topics include generating synthetic data for survey data with sampling weights, and developing the framework for computer systems that provide secondary analysts with feedback on the quality of inferences from heavily redacted data.
NISS and Duke are also working with Cornell University as the coordination office for the National Census Research Network (NCRN-CO). NISS hosts the NCRN.Info website.
Develop methodology to improve current practice for handling missing and faulty data and statistical disclosure limitation.
Principal Investigator(s): Jerry Reiter, Duke; Alan Karr, Co-PI, NISS/RTI
Senior Investigators: Lawrence Cox, NISS; David Dunson, Duke; D. Sunshine Hillygus, Duke; V. Joseph Hotz, Duke; Fan Li, Duke; Seth Sanders, Duke; Rebecca Steorts, Duke
Postdoctoral Fellows: Hang Kim, Neung Soo Ha, Mauricio Sadinle