Research Project
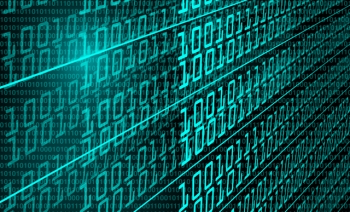
NISS conducted research in data confidentiality, data quality, and data integration. Prototypes were built which could scale to operate on large sets of Federally held data. Researchers partnered with several large Federal Government statistical agencies. This topic was of particular importance given the balance of these agencies must strive for, in terms of their dual missions to collect and keep private confidential data, while at the same time making that data accessible for research and policy issues. NISS helped convene a multi-disciplinary multi-institution team, with participants from five universities, one non-profit, and one national laboratory. The disciplines represented include computer science, statistical science, and systems engineering.
Technical Report(s):
Technical Report 124: A Divide-and-Conquer Algorithm for Generating Markov Bases of Multi-way Tables
Technical Report 125: Software Systems for Tabular Data Releases
Technical Report 126: NISS WebSwap: A Web Service for Data Swapping
Technical Report 129: Data Quality: A Statistical Perspective
Technical Report 130: Preserving Confidentiality of High-dimensional Tabulated Data: Statistical and Computational Issues
Technical Report 131: Distortion Measures for Categorical Data Swapping
Technical Report 132: A Risk-Utility Framework for Categorical Data Swapping
Technical Report 134: Data Swapping: A Risk-Utility Framework and Web Service Implementation
Technical Report 138: Data Dissemination and Disclosure Limitation in a World Without Microdata: A Risk-Utility Framework for Remote Access Analysis Servers
Technical Report 140: Data Swapping as a Decision Problem
Technical Report 141: Secure Regression on Distributed Databases
Technical Report 142: Database Security and Confidentiality: Examining Disclosure Risk vs. Data Utility through the R-U Confidentiality Map
Technical Report 143: Privacy Preserving Regression Modelling via Distributed Computation
Technical Report 145: Privacy Preserving Analysis of Vertically Partitioned Data Using Secure Matrix Products
Technical Report 146: Secure Regression for Vertically Partitioned, Partially Overlapping Data
Technical Report 147: Secure Statistical Analysis of Distributed Databases
Technical Report 149: Data Quality and Data Confidentiality for Microdata: Implications and Strategies
Technical Report 151: Data Quality: A Statistical Perspective
Technical Report 152: Secure Analysis of Distributed Chemical Databases without Data Integration
Technical Report 153: A Framework for Evaluating the Utility of Data Altered to Protect Confidentiality
Technical Report 158: Secure, Privacy-Preserving Analysis of Distributed Databases
Technical Report 160: Secure computation with horizontally partitioned data using adaptive regressive splines
Technical Report 171: Bayesian Multiscale Multiple Imputation with Implications to Data Confidentiality
Technical Report 179: Risk-Utility Paradigms for Statistical Disclosure Limitation: How to Think, But Not How to Act
Funding Sponsor: National Science Foundation
Principal Investigator(s): Alan Karr, NISS; Stephen Fienberg, Carnegie Mellon
Senior Investigator(s): Jerry Reiter, Duke, David Banks, Duke
Post Doctoral Fellow(s): Adrian Dobra, Ashish Sanil, Shanti Gomatam, Xiaodong Lin