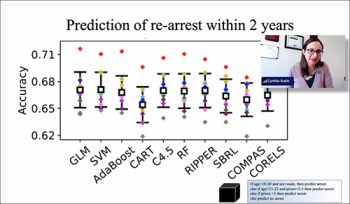
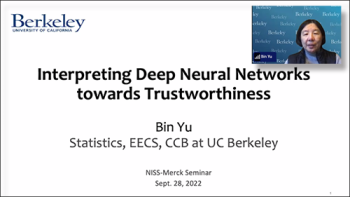
The National Institute of Statistical Sciences (NISS) and Merck sponsored a NISS/Merck Meetup on Interpretable/Explainable Machine Learning on Wednesday, September 28, 2022 from 11:30am-1:00pm ET. (see Event page)
Interpretable/explainable Machine Learning (ML) is an emerging field to address the black-box nature of complex models obtained by many popular ML methods. Its success can remove one of the major obstacles that prevent ML from having more impact on areas such as healthcare, where human understanding of how a data-driven model works is crucial for many reasons. As many research fields have experienced in their infancy, few widely accepted notions and boundaries have given researchers the freedom to formulate this field with their visions, and to define the key concepts, such as interpretability and explainability, in their own ways. In this meetup, two leading researchers shared their efforts, contributions, and visions about this rapidly developing field.
The speakers included Professor Cynthia Rudin from Duke University and Professor Bin Yu from the University of California, Berkeley. The moderator for this session was Junshui Ma from Merck. Each of our speakers gave a 35-minute talk, with a very hefty live Q&A session at the end.
Our first speaker of the day was Cynthia Rudin, with her presentation "Do Simpler Machine Learning Models Exist, and How Can We Find Them?" Cynthia is currently a professor of four different departments at Duke University including Computer Science, Electrical and Computer Engineering, Statistical Science and Biostatistics and Bioinformatics. She also directs the Interpretable Machine Learning Lab at Duke. Cynthia started working in the field of ML because she was working with a New York City power company trying to predict power liability issues. Through her work, she realized that complex machine learning models were not getting her any better predictions than very simple models. She had thought that maybe the problem that she was working on was an anomaly, but it wasn’t; the same thing kept happening again and again.
Cynthia provided an example of an article she wrote with others called “Interpretable classification models for recidivism prediction.” In this article they used the largest public dataset of criminal recidivism on 33k individuals that were all released from prison in the same year. Using interpretable ML to predict whether someone would commit different types of crimes after they were released from prison, it was concluded that a complicated model is not needed to predict recidivism, and that race is not a useful variable. Cynthia along with her team did a comparison on an article on COMPAS (a proprietary model used in the justice system using race) with CORELS which uses quite simple models. The accuracy between the two were similar, but they questioned if they could do any better than this. Even from using many different types of proprietary models, they still came out with similar results.
Her next example is based on preventing brain damage in critically ill patients, where EEG is needed to detect seizures. Cynthia helped design the 2HELPS2B score which is a simple model that doctors could use much faster than EEG. The doctors can memorize the model just by knowing its name! This model was designed by an algorithm for interpretable machine learning where the selection of features and the point scores get chosen by the algorithm.
“It is just as accurate as black box models on the dataset, it led to a substantial amount of reduction of the duration of EEG monitoring per patient, which allowed the doctors to monitor a lot more patients and reduce brain damage and save lives!”
- Cynthia Rudin
Is there really a benefit of complex models for a lot of problems? Doesn’t seem like it, but yet we’re often using complicated or proprietary models for high stakes societal decisions when we don’t actually need them if we can determine the same results from simple models.
Bin Yu’s presentation was entitled, "Interpreting deep neural networks towards trustworthiness." Professor Bin is currently the Chancellor's Distinguished Professor and Class of 1936 Second Chair in the Departments of Statistics and EECS, and Center for Computational Biology at UC Berkeley. She has published in over 170 publications in premier venues.
Bin emphasized that the data science is a cyclical process and that interpretation is an important part of this process. This led to the investigation of the role of relevancy or a reality check with regards to interpretable machine learning to determine whether certain models are viable or not. She then walked through a couple of examples that demonstrate this concept. Her next point involved the introduction of trust in interpretable machine learning. During rest of her talk Bin shread three extensive out of context approaches where applying a new context sensitive approach to the model interpretation improved the prediction results.
"What approach do you prefer? Out of contenxt or in context? ... I always prefer in context because the reality check in solving a particular problem is really powerful and actually make it more likely to be useful."
Bin Yu
Junshui Ma (Merck) the moderator and organizer for this session then entertained a couple of questions from the viewers.
Recording of this Session