Distributionally Robust Two- and Multi-Stage Stochastic Programming
Speaker
David Morton, Northwestern University
Abstract
Distributionally robust optimization allows decisions from a stochastic optimization model to hedge against a range of distributions, characterized by an ambiguity set. We first study two-stage stochastic programs with linear recourse and formulate distributionally robust models that vary in how the ambiguity set is built, using the Wasserstein distance and an optimal quadratic transport distance. We consider both unbounded and bounded support sets, and provide guidance regarding which models are meaningful in the sense of yielding robust first-stage decisions. Second, we consider a multi-stage stochastic linear program that lends itself to solution by stochastic dual dynamic programming (SDDP). In this context, we again consider a distributionally robust variant of the model. We describe a computationally tractable variant of SDDP to handle this model using the Wasserstein distance. This is joint work with Daniel Duque and Sanjay Mehrotra.
Bio
David Morton is the David A. and Karen Richards Sachs Professor and Department Chair in Industrial Engineering and Management Sciences at Northwestern University. Prior to joining Northwestern, he served on the faculty at the University of Texas at Austin and as a postdoctoral fellow at the Naval Postgraduate School. He research interests include stochastic optimization for decision making under uncertainty. He worked as a Fulbright Scholar at Charles University in Prague, was a finalist for the EURO Excellence in Practice Paper Prize, and is an INFORMS fellow.
Event Type
- NISS Sponsored
Sponsor
Cost
Website
Location
Policy
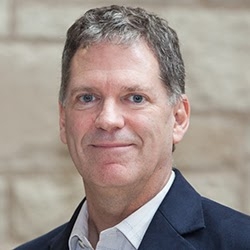