Robust Online Learning and its Applications to Assortment Optimization
Speaker
Xi Chen (New York University)
Abstract
In this talk, we will first provide an overview of my research on online learning and decision-making. We will highlight a few applications on dynamic pricing, assortment optimization for online recommendation, and crowdsourcing.
Most online learning problems are built on an underlying probabilistic model. However, these models are inherently misspecified to a certain degree, which calls for robust learning methods. The second part of the talk will discuss robust online learning and its applications to recommendations. In particular, we consider the dynamic assortment optimization problem under the multinomial logit model (MNL) with unknown utility parameters. Based on online eps-contamination modeling of customers’ purchase behavior, we develop a rate-optimal robust online assortment optimization policy via an active elimination strategy.
Bio
Xi Chen is an associate professor at Stern School of Business at New York University, who is also an affiliated professor to Computer Science and Center for Data Science. Before that, he was a Postdoc in the group of Prof. Michael Jordan at UC Berkeley. He obtained his Ph.D. from the Machine Learning Department at Carnegie Mellon University. He studies high-dimensional statistical learning, online learning, large-scale stochastic optimization, and applications to revenue management and crowdsourcing. He received NSF Career Award, Outstanding Faculty Research Awards from Google, Facebook, Adobe, Alibaba, and Bloomberg, JPMorgan, and was featured in Forbes list of “30 Under 30 in Science
Event Type
- NISS Sponsored
Sponsor
Website
Location
Policy
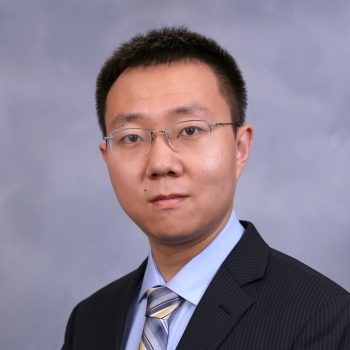