Preconditioning Helps: Faster Convergence in Statistical and Reinforcement Learning
Speaker
Yuejie Chi, (Carnegie Mellon University)
Abstract
Exciting progresses have been made in demystifying the efficacy of vanilla gradient methods in solving challenging nonconvex problems in statistical estimation and machine learning. In this talk, we discuss how the trick of preconditioning further boosts the convergence speed with minimal computation overheads through two examples: low-rank matrix estimation in statistical learning and policy optimization in entropy-regularized reinforcement learning. For low-rank matrix estimation, we present a new algorithm, called scaled gradient descent, that achieves linear convergence at a rate independent of the condition number of the low-rank matrix at near-optimal sample complexities for a variety of tasks. For policy optimization, we develop the first fast non-asymptotic convergence guarantee for entropy-regularized natural policy gradient methods in the tabular setting for discounted Markov decision processes. By establishing its global linear convergence at a near dimension-free rate, we provide theoretical footings to the empirical success of entropy-regularized natural policy gradient methods. Based on arXiv 2005.08898 and 2007.06558.
Bio
Yuejie Chi is an Associate Professor in the Dept. of Electrical and Computer Engineering at Carnegie Mellon University, where she holds the Robert E. Doherty Career Development Professorship. She is also an affiliated faculty member with the Machine Learning Department and CyLab.
Her research interests lie in the theoretical and algorithmic foundations of data science, signal processing, machine learning and inverse problems, with applications in sensing systems, broadly defined. She uses tools at the intersection of high-dimensional statistics, convex and nonconvex optimization, information and sampling theory.
She received Presidential Early Career Award for Scientists and Engineers (PECASE) from the White House, NSF CAREER Award, AFOSR YIP Award, ONR YIP Award, Google Faculty Research Award, IEEE Signal Processing Society Young Author Best Paper Award, among others. In 2019, She received the inaugural IEEE Signal Processing Society Early Career Technical Achievement Award for contributions to high-dimensional structured signal processing.
Previously she was with the Dept. of Electrical and Computer Engineering and the Dept. of Biomedical Informatics at The Ohio State University until 2017. She completed my Ph.D. in Electrical Engineering from Princeton University in 2012, where I was fortunate to be advised by Prof. Robert Calderbank. She received a M.A. in Electrical Engineering from Princeton University in 2009, and a B.Eng. in Electronic Engineering from Tsinghua University in 2007.
Event Type
- NISS Sponsored
Sponsor
Website
Location
Policy
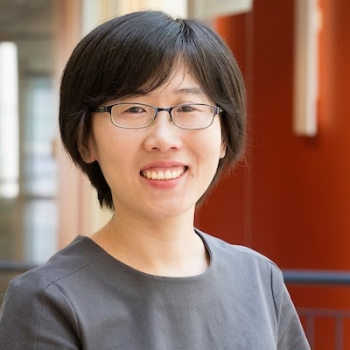