Convergence and Sample Complexity of Gradient Methods for the Model-Free Linear Quadratic Regulator Problem
Abstract
Model-free reinforcement learning attempts to find an optimal control action for an unknown dynamical system by directly searching over the parameter space of controllers. The convergence behavior and statistical properties of these approaches are often poorly understood because of the nonconvex nature of the underlying optimization problems and the lack of exact gradient computation. In this talk, we discuss performance and efficiency of such methods by focusing on the standard infinite-horizon linear quadratic regulator problem for continuous-time systems with unknown state-space parameters. We establish exponential stability for the ordinary differential equation (ODE) that governs the gradient-flow dynamics over the set of stabilizing feedback gains and show that a similar result holds for the gradient descent method that arises from the forward Euler discretization of the corresponding ODE. We also provide theoretical bounds on the convergence rate and sample complexity of the random search method with two-point gradient estimates. We prove that the required simulation time for achieving $\epsilon$-accuracy in the model-free setup and the total number of function evaluations both scale as $\log (1/\epsilon)$.
Bio
Mihailo R. Jovanovic is a professor in the Ming Hsieh Department of Electrical and Computer Engineering and the founding director of the Center for Systems and Control at the University of Southern California. He was a faculty member in the Department of Electrical and Computer Engineering at the University of Minnesota, Minneapolis, from December 2004 until January 2017, and has held visiting positions with Stanford University and the Institute for Mathematics and its Applications. His current research focuses on large-scale and distributed optimization, design of controller architectures, dynamics and control of fluid flows, and fundamental limitations in the control of large networks of dynamical systems. He serves as an Associate Editor of the IEEE Transactions on Control of Network Systems and had served as a Guest Editor (of the Special Issue on Analysis, Control and Optimization of Energy System Networks in the IEEE Transactions on Control of Network Systems), the Chair of the APS External Affairs Committee, a Program Vice-Chair of the 55th IEEE Conference on Decision and Control, an Associate Editor of the SIAM Journal on Control and Optimization, and an Associate Editor of the IEEE Control Systems Society Conference Editorial Board. Prof. Jovanovic is a fellow of APS and IEEE. He received a CAREER Award from the National Science Foundation in 2007, the George S. Axelby Outstanding Paper Award from the IEEE Control Systems Society in 2013, and the Distinguished Alumnus Award from UC Santa Barbara in 2014.
Event Type
- NISS Sponsored
Sponsor
Website
Location
Policy
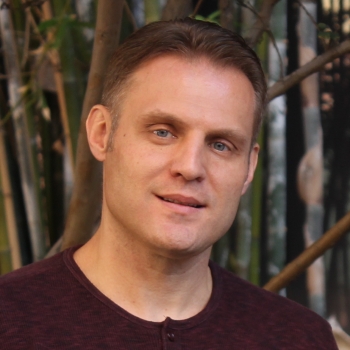