Nonparametric Active Learning with Kernels and Neural Networks
Speaker
Robert Nowak, University of Wisconsin, Madison
Abstract
The field of Machine Learning (ML) has advanced considerably in recent years, but mostly in well-defined domains using huge amounts of human-labeled training data. Machines can recognize objects in images and translate text, but they must be trained with more images and text than a person can see in nearly a lifetime. The computational complexity of training has been offset by recent technological advances, but the cost of training data is measured in terms of the human effort in labeling data. People are not getting faster nor cheaper, so generating labeled training datasets has become a major bottleneck in ML pipelines.
Active ML aims to address this issue by designing learning algorithms that automatically and adaptively select the most informative examples for labeling so that human time is not wasted labeling irrelevant, redundant, or trivial examples. This talk explores the development of active ML theory and methods over the past decade, including a new approach applicable to kernel methods and neural networks, which views the learning problem through the lens of representer theorems. This perspective highlights the effect that adding a given training example has on the representation. The new approach is shown to possess a variety of desirable mathematical properties that allow active learning algorithms to learn good classifiers from few labeled examples.
Bio
Robert Nowak is the McFarland-Bascom Professor in Engineering at the University of Wisconsin-Madison, where his research focuses on signal processing, machine learning, optimization, and statistics. He is a professor in Electrical and Computer Engineering, as well as being affiliated with the departments of Computer Sciences, Statistics, and Biomedical Engineering. He is a Fellow of the IEEE and the Wisconsin Institute for Discovery, a member of the Wisconsin Optimization Research Consortium, and Machine Learning@Wisconsin, and is an organizer of the SILO seminar series. He is also an Adjoint Professor at the Toyota Technological Institute at Chicago.
Event Type
- NISS Sponsored
Sponsor
Website
Location
Policy
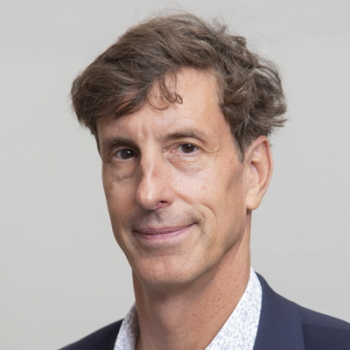