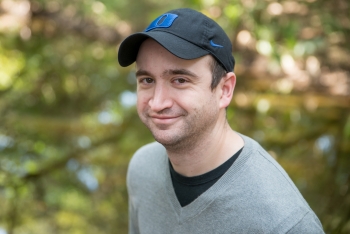
Born and raised in Greenville, South Carolina, Michael Robbins was always interested in quantitative research. He went to high school at JL Mann Academy in Greenville, South Carolina. He earned his bachelor’s degree in Mathematics and Economics from Duke University in Durham, North Carolina, and then went on to earn his master’s degree in Mathematical Sciences from Clemson University. Robbins also pursued a Ph.D. degree in Mathematical Sciences from Clemson University and received his degree in 2009. Immediately following his Ph.D., he joined NISS as a Postdoctoral fellow from 2009 to 2011.
Robbins always had a passion for statistics. He enjoyed probability theory and has always been quantitatively inclined. “Probability theory was something that clicked for me in high school and college. I took some statistics in high school and college, but found it rather enigmatic," says Robbins. He goes on to say, "You were taught how to perform an F-test, use a T-table, etc., with no idea of how or why any of it works. It wasn’t until I took mathematical statistics in grad school and learned the underpinnings of all the methodology that I really started to enjoy statistics."
Robbins owes his successes to his mentors who he says played an important role guiding him all through his career. His Ph.D. advisor from Clemson University, Colin Gallagher, was proud to see Robbins transform from a student to an independent researcher while at Clemson. "Michael was great to work with," says Gallagher. Sharing his experiences working with Robbins, Gallagher says that Robbins showed up for research meetings with a candy bar and a soda, and he was always ready to dig in and talk mathematics. He enjoyed going to the whiteboard and deriving things on the spot. He liked to prove and explain the methodologies and the theoretical results, and most importantly, he constantly improved himself as a researcher. “If he didn't understand or know something he would go study it until he mastered it, then come back and explain it in a research meeting,” says Gallagher.
Gallagher guided his mentees on how to formulate and attack a research problem, how to write a research paper, and how to give a research talk. As Robbins’ mentor for four years, Gallagher remembers listening to Robbins practice research talks and guiding him through the process. "Initially we had a mentor-mentee relationship but by the end, I was able to treat Michael as a collaborator rather than a student researcher," says Gallagher.
As a Postdoc at NISS, Robbins developed an imputation algorithm for the USDA's Agricultural Resource Management Survey (ARMS). ARMS are a high-dimensional survey of more than 1000-2000 questions asked to 30,000-40,000 respondents who in this project were farmers from Iowa, Illinois, and other agricultural producing states. An imputation method is used to address the missing values because respondents don't usually answer all questions in the survey. This project titled, "Imputation in High-Dimensional Economic Data as Applied to the Agricultural Resource Management Survey” was administered by NISS and the National Agricultural Statistical Service (NASS). “It means that I have multiple numbers of items in the questionnaire that have multiple types of missing values and I want to impute those missing values using statistical methodology... that's exactly what we developed,” says Dr. Sujit Ghosh, Professor at NC State University, Department of Statistics who mentored Robbins on this project.
Sharing his experience working with Robbins, Dr. Ghosh says, "Michael has the capability of taking on new projects. When he joined the program, he was not an expert on the methodology of imputation. He didn't have that background, but he worked hard, picked up the literature, read it thoroughly, and not only that, he came up and shared some creative research ideas too. He has the capability of picking up a topic and working on it, even if he has no background in the subject."
Speaking about the challenges of moving from school to a real-job at NISS, Robbins says, "You’re accustomed to working under heavy guidance in school and now have to adapt to working largely on your own. The biggest adjustment for me from after my postdoc experience was learning to juggle many research projects simultaneously."
Prior to Robbins work at NISS, another Ph.D. advisor from Clemson University, Robert Lund, was happy to mentor Robbins for more than four years. All Lund had to do was give Robbins a technical problem to challenge him. Robbins would come back with the answer to the problem the very next day. "He’s a great abstract thinker and an astute student capable of so much," says Lund.
Robbins is now a statistician at RAND Corporation and has been extensively working on surveys. He was instrumental in building RAND's American Education Panels which are survey panels of over 30,000 educators. Robbins also uses his broad statistical toolkit and applies it to different areas such as health, education, criminology, military, defense, including others.
Robbins is working on an R package called "Microsynth" in collaboration with Steve Davenport, Assistant Policy Researcher at RAND Corporation. Davenport is also a doctoral candidate at the Pardee RAND Graduate School and Robbins is mentoring Davenport on this project. Microsynth is a product of a RAND Corporation project that aims to improve the matching of treatments to synthetic controls and to construct better estimators for the variance within synthetic control methods.
The synthetic control method is a statistical method used to evaluate the effect of an intervention in comparative case studies. There are many research studies that have applied the synthetic control methods, and there are packages already available in languages such as R that facilitate the use of synthetic controls. But the existing packages have built-in limitations that were appropriate in a relatively data-scarce environment, with a small number of unit-observations.
With the arrival of Big Data, "Microsynth is built to better take advantage of big data and counter these limitations,” says Davenport, adding, "Microsynth exploits an abundance of data to improve the matching of different treatments and synthetic controls. Additionally, it also enhances the method by adapting the algorithms for use in data-rich environments, so that research can be conducted on many micro-level observational units."
Speaking about working with Robbins on the Microsynth project, Davenport says, "Michael has been invaluable as a mentor and partner: tremendously responsive and knowledgeable. That he has developed such a methodology is impressive and makes the rest of the project much easier."