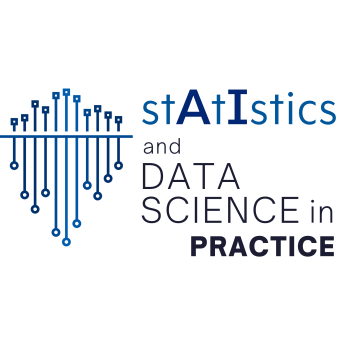
Webinar Date: Friday, January 24, 2025
The NISS AI, Statistics and Data Science in Practice Webinar: Victor Lo & Victor Chen - Causal AI in Business Practices focused on causal inference techniques and their applications in various fields, including business, social sciences, and medicine. The speakers discussed different approaches to causal analysis, such as the Statistical Approach, Pearl Causal Model, and double machine learning, as well as their limitations and potential for automation through AI. The presenters also explored the relationship between AI, machine learning, and causal reasoning, highlighting the importance of understanding causal relationships in decision-making processes and policy evaluations.
Nancy welcomed participants to the NISS Webinar series on AI statistics and data science. She introduced the speakers, Dr. Victor Lo and Dr. Victor Chen, both with extensive experience in data science and AI. Dr. Lo discussed the importance of causal inference techniques, which have applications across various fields including customer relationship management, marketing, and risk management. He also mentioned the recent Turing and Nobel awards related to causality. Dr. Chen was expected to share his insights on the topic.
Causality in Business and Social Sciences
Victor Lo discussed the importance of causality in various fields, including business, social sciences, and medicine. He highlighted the need to understand the impact of interventions, such as policy changes or marketing efforts, and to answer counterfactual questions. He also emphasized the importance of explanation, tracing the causal mechanisms that lead to outcomes. Victor then provided examples from the business world, focusing on marketing strategies like price, promotion, location, and product features. He also introduced the concept of randomized control trials (RCTs) as the gold standard for measuring causality. Lastly, he mentioned the Rubin Causal Model (RCM) and propensity score matching, which are widely used in statistics, social sciences, and medicine.
Causal Inference Approaches and Applications
Victor Lo discussed two approaches to causal inference: the Statistical Approach and the Pearl Causal Model. The Statistical Approach, also known as propensity score matching, involves statistical adjustments to handle confounders and infer missing data. It is widely used in the business world for assessing the impact of sales efforts on customer retention. The Pearl Causal Model, on the other hand, relies on causal graphs and the Markov condition theory to estimate joint distributions of variables. It is less known in the statistics community but has applications in AI and computer science. Victor also illustrated the use of these methods with a study on the relationship between health and finance, where the direction of causality was found to be bidirectional.
Pearl Approach to Causal Analysis
Victor Lo discussed the Pearl approach to causal analysis, which involves constructing a deck based on domain knowledge, collecting data, and using structural learning algorithms to identify causal relationships. He highlighted the importance of controlling for confounders and estimating treatment effects. Victor also addressed questions about the frequency of correlation not equaling causation and the applicability of the Pearl causal model without domain expertise. He provided a practical example of applying the Pearl approach in the business world, emphasizing the importance of understanding direct and indirect impacts of interventions.
Causal Discovery and Structural Modeling
Victor S.Y. discussed the relationship between structural equation modeling and Pearl's approach, highlighting the key difference as Pearl's approach can recommend a graph even with little or no idea of the graph's structure. He also addressed the limitations of causal discovery approaches in handling hidden confounders, stating that domain knowledge is essential. Victor S.Y. provided an example of a confounder in the business world, using historical sales data and customer contact information. He recommended the 'do' package for causal discovery in Python and clarified the interpretation of bidirectional relationships in a DAG. Lastly, he briefly touched on the economic approach to causal inference, mentioning the 'difference in difference' method as a basic causal inference method.
Measuring Policy Effectiveness and Optimization
Victor S.Y. discussed various methods for measuring the effectiveness of policy changes and interventions. He explained the parallel trend assumption, the synthetic control method, and the use of instrumental variables. He also discussed the potential for combining different methods to improve accuracy. Victor highlighted the importance of understanding the individual treatment effect, particularly in marketing and political campaigns, and the potential for optimizing outcomes by targeting specific demographics. He concluded by mentioning the use of uplift models in political campaigns and the importance of considering the potential harm of interventions.
AI, Machine Learning, and Causal Reasoning
Victor Chen discusses the relationship between AI, machine learning, and causal reasoning. He sees AI as an overarching concept aimed at imitating human cognitive functions, with machine learning and causal reasoning as subsets that often overlap. The presentation focuses on this overlapping area between machine learning and causal reasoning. Chen covers reinforcement learning for experimentation, causal inference, causal discovery, causal machine learning, causal language models, and eventually agent AI combining all these capabilities. He explains how reinforcement learning algorithms like multi-armed bandits balance exploration and exploitation to maximize rewards over time. Chen then uses the example of personalized recommendations on platforms like YouTube, Google News, and Netflix to illustrate how reinforcement learning optimizes user experiences through iterative trial and error.
Traditional vs Modern Bandits Testing
Victor Chen discussed the differences between traditional A/B testing and modern bandits testing. He explained that traditional A/B testing involves randomly splitting traffic into different groups, waiting for a significant sample size, and then rolling out the best-performing variant. However, this method can be inefficient as it may hurt a large portion of potential customers. In contrast, modern bandits testing dynamically adjusts the exposure of different variants over time, allowing for more efficient allocation of traffic. Victor also introduced contextual bandits, which aim to personalize the allocation of different variants based on audience characteristics. He noted that while reinforcement learning offers flexibility, it has limitations, such as requiring frequent responses and sufficient sample sizes.
Causal Reasoning and Machine Learning
Victor Chen discussed the limitations of AI and causal reasoning in various applications. He clarified that once validation for a causal model is built, it doesn't require any causal model as it's randomized. He also explained the difference between causal inference and causal discovery, stating that the former requires domain knowledge to assume or build a structure model graph connecting causal directions among different variables, while the latter tries to represent the directions of causal relationships between different variables. Victor also touched on the topic of causal machine learning, focusing on double machine learning, a branch of causal machine learning that uses machine learning to analyze observational data to estimate causal effects. He mentioned that this approach has been around for about 10 years and is used to estimate the causal effects of treatments and structural parameters. Nancy asked about the validation of a causal model and how Thompson sampling handles small traffic over time in multi-armed bandits, to which Victor responded that Thompson sampling is used as one mechanism of randomizing traffic but there could be many others.
Exploring Double Machine Learning Method
Victor Chen discussed a method called double machine learning, which involves predicting and removing the predictable part of a variable (D) based on another variable (Z). He explained that this method can be used to analyze the relationship between the residuals of these predictions and infer the causal impact of D on another variable (Y). He also mentioned that this method can be applied to longitudinal data where confounders cannot be observed. Victor provided two examples of how this method could be used: to determine if the popularity of a content (like Squid Game) is causally driving user engagement, and to assess the long-term return on investment. He emphasized that by removing the predictable portion of the treatment, the method can help infer causality between variables.
Machine Learning and Causal Modeling
Victor Chen discussed the limitations of machine learning and causal modeling, particularly in the context of observational studies. He highlighted that machine learning cannot account for unmeasured confounders and that causal models are not perfect. He also mentioned the potential for AI to automate causal reasoning and decision-making processes, but noted that this would require human feedback at each stage. Nancy asked about the provision of uncertainty interval estimates for average treatment effects in double machine learning, to which Victor responded affirmatively. The conversation ended with Nancy expressing appreciation for the speakers' insights and the end of the session.