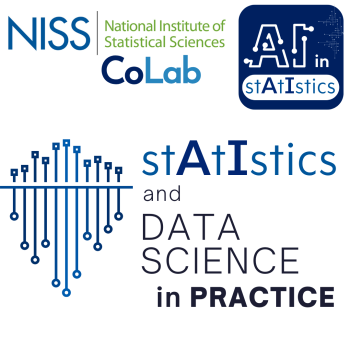
News Story: Tuesday, November 19, 2024 | 12:00 PM - 1:30 PM ET
In the latest session of the NISS AI, Statistics & Data Science in Practice series, Dr. Lucas Mentch, Associate Professor of Statistics at the University of Pittsburgh, delivered a thought-provoking talk titled Random Forests: Why They Work and Why That’s a Problem. The session, moderated by Nancy McMillan, a data science leader at Battelle and Chair of the NISS Affiliates Committee, provided a deep dive into the challenges and potential solutions in using machine learning models, with a focus on Random Forests. Nancy McMillan opened the session by introducing Dr. Mentch, whose work bridges machine learning, statistical inference, and theory. With applications ranging from medicine to forensic science, Dr. Mentch’s insights aimed to demystify the inner workings of Random Forests, addressing both their strengths and inherent challenges.
The Enigma of Random Forests
Dr. Mentch began his presentation by outlining the fundamental appeal of Random Forests. As one of the most widely used machine learning algorithms, Random Forests have consistently delivered strong predictive performance across a wide range of applications. He cited a 2014 comparative study that ranked Random Forests as the top-performing classifier among dozens of algorithms. Despite their effectiveness, the underlying reasons for their success remain incompletely understood. One key theme was the trade-off between the randomness introduced during model construction and the complexity of natural phenomena that Random Forests aim to capture. Dr. Mentch critiqued the commonly held explanations for their performance, such as the accuracy-correlation trade-off, arguing that these are more motivational rather than explanatory.
Challenges of Complexity and Transparency
The primary issue Dr. Mentch highlighted was the opacity of Random Forest models. Their intricate structures make it difficult to derive statistically valid inferences about variable importance or predictive reliability. To address this, Dr. Mentch proposed replacing the bootstrapping step of Random Forests with subsampling. This subtle yet impactful adjustment allows for the development of confidence intervals and hypothesis tests, enhancing interpretability without sacrificing performance. In a case study involving data on indigo buntings, Dr. Mentch illustrated the risks of model over-reliance on noise features. Even when the variable for "month" was randomized, the model returned significant results, underscoring the dangers of misinterpreting noise as meaningful information. To combat this, he advocated for methods such as knockoffs and permutation tests, which can more accurately assess variable significance.
Randomness and Regularization
A recurring topic was the interplay between randomness and regularization. Dr. Mentch discussed how adding randomness during the model-building process can implicitly regularize the model, reducing overfitting in noisy data scenarios. He also explored the implications of augmented bagging, a technique where random noise features are deliberately added to the dataset. While this approach can sometimes improve model performance, it raises concerns about the validity of inferences, particularly in high-dimensional datasets.
Applications and Future Directions
Dr. Mentch connected his findings to a variety of domains, including time series analysis and ecological modeling. Responding to questions from Ms. McMillan, he explained how Random Forests treat time-series data as independent observations and how their competitive performance persists even in the era of deep learning. Looking ahead, Dr. Mentch emphasized the importance of understanding the relationships between noise features and genuine predictors. He shared preliminary results suggesting that Random Forests outperform other methods in noisy data environments but may lose their edge as data quality improves. This observation has significant implications for practitioners selecting models in real-world applications.
A Vibrant Discussion
The session concluded with an engaging Q&A. Nancy McMillan skillfully facilitated a dialogue that touched on advanced topics such as the role of shrinkage via noise, the application of Ridge regression in tree-based models, and the integration of Random Forests within deep learning architectures. Dr. Mentch expressed optimism about the continued evolution of machine learning tools while cautioning against their misuse in high-dimensional, noisy data settings. Nancy closed the session by thanking Dr. Mentch and the audience, reminding attendees that the slides and recording would soon be available on the NISS website and YouTube channel.
Thanks and Recognition
NISS and the Affiliates Committee thanks Dr. Lucas Mentch for giving a presentation that illuminated both the potential and the pitfalls of Random Forests, providing attendees with valuable insights into one of the most robust tools in machine learning. By bridging theoretical advancements with practical applications, this session exemplified the mission of NISS to foster dialogue and innovation at the intersection of AI, data science, and statistics.
Resources