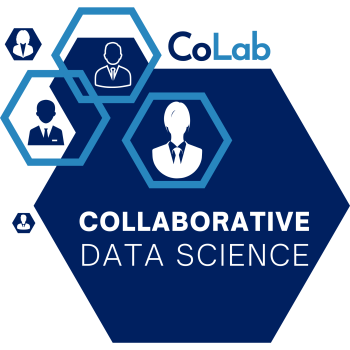
Date: Thursday, April 10, 2025, at 1–2 pm ET
Part of the Collaborative Data Science series organized by NISS and the Canadian Statistical Sciences Institute (CANSSI), this webinar focused on interdisciplinary approaches to complex data challenges in astronomy and cosmology. The speakers discussed the creation of cosmic maps, the use of simulations and emulation tools to study the universe's composition and evolution, and methods for calibrating cosmological models. They emphasized the importance of statistical analysis and large-scale simulations in interpreting observational data and constraining cosmological parameters.
NISS and Canadian Statistical Sciences Institute's Collaborative Data Science Webinar Series
As the moderator for this webinar, Emily Castleton, Chair of the NISS-CANSSI Collaborative Data Science Committee and based at Los Alamos National Lab, introduced the joint NISS and Canadian Statistical Sciences Institute's collaborative data science webinar series, which aims to showcase experts tackling complex data challenges through interdisciplinary teamwork. She thanked the National Institute for Statistical Sciences and the Canadian Statistical Sciences Institute for sponsoring the series. Emily also announced the next webinar on AI for health data and encouraged attendees to submit ideas for future webinars. She then introduced the speakers, Dr. Kelly Moran and Dr. Katrin Heittman, who would discuss astronomy and cosmic emulation. Emily emphasized the importance of putting questions in the Q&A for the speakers to address during the presentation.
Cosmology: Mapping the Universe's Composition
Katrin provides an introduction to cosmology, focusing on the creation of maps to observe and understand the universe. She explains that 95% of the universe consists of dark energy (69%) and dark matter (26%), which are not fully understood. Katrin discusses ongoing projects like the Rubin Observatory and DESI, which are creating detailed maps of the cosmos. She emphasizes the importance of statistical analysis and large-scale simulations in interpreting these maps to answer fundamental questions about the universe's composition and evolution.
Cosmic Emulation: Accurate Matter Power Spectrum
Katrin and Kelly discussed the use of simulations in cosmology, focusing on the matter power spectrum. Kelly presented a tool called the Cosmic Emu, which emulates the power spectrum for various cosmological parameters. The tool was shown to be highly accurate, with a predictive performance of less than 1% error. Kelly also explained the process of running simulations, including the use of nested space filling design and the combination of theoretical and simulation results. The team is now working on refining the tool and exploring its potential applications.
Gaussian Process Modeling With Sepia
Kelly discussed the foundation of a model originally published by Dave Higden, which involves centering, scaling data, projecting it onto an orthogonal basis, and modeling the basis weights with Gaussian processes. Kelly explained that for each basis weight, a Gaussian process is used to model it, and this is wrapped up in a Bayesian model that learns the behaviors of the Gaussian process and the precision of the overall principal component representation. Kelly also explained that a Gaussian process is a way to represent multivariate data as a multivariate normal distribution, and it can describe the relationship between any two observations using a correlation function. Kelly mentioned that the Gaussian process is implemented in Python as sepia, which was a more user-friendly package than the original MATLAB implementation.
Cosmological Simulation Calibration and Emulation
Kelly and Katrin present a method for emulating and calibrating cosmological simulations. They use principal component analysis to decompose the power spectrum of gravity-only simulations across different cosmological parameters. This allows them to accurately predict power spectra for new cosmologies. They then incorporate additional astrophysics like gas physics and star formation using subgrid models with 5 parameters. By varying these parameters and comparing to observational data on galaxy stellar mass, gas fraction, and density, they can constrain the most likely parameter values. Using multiple observables together provides tighter constraints than individual observables alone. The calibrated model allows them to run larger, higher-resolution hydrodynamical simulations with plausible parameters.
Acknowledgements
NISS and CANSSI extend their heartfelt thanks to speakers Dr. Kelly Moran and Dr. Katrin Heittman for their outstanding presentations and contributions to advancing interdisciplinary work in cosmology and data science. Special appreciation goes to Emily Castleton for her leadership as moderator and for her ongoing role in shaping the NISS-CANSSI Collaborative Data Science Webinar Series. Their combined efforts made this event both informative and inspiring to the data science and astronomy communities.
About the NISS-CANSSI
Collaborative Data Science Web Series:
The NISS-CANSSI Collaborative Data Science initiative that the National Institute of Statistical Sciences (NISS) in collaboration with the Canadian Statistical Sciences Institute (CANSSI) brings together experts from various fields to tackle complex data challenges through interdisciplinary teamwork and innovative methodologies.
Goals of the Initiative
The goal is to foster progress in:
- Developing new ideas for experimental and observational data-driven learning and discovery that address key questions at the cutting edge of science and scientific deduction;
- Quantifying and summarizing uncertainty in data-driven theories, as well as complex Data Science models, algorithms, and workflows; and
- Establishing new practices for scientific reproducibility and replicability through Data Science.
Featured Webinars
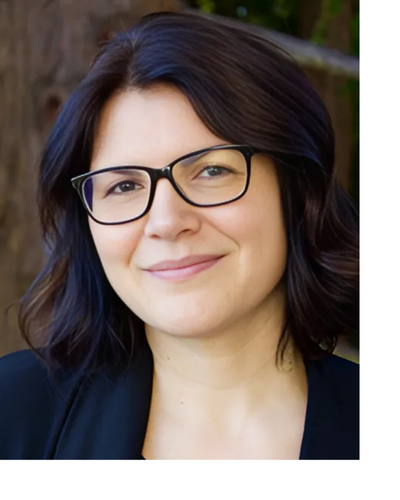
Changing Climate, Changing Data: A journey of statisticians and climate scientists
Date: Thursday, March 20, 2025 at 1-2pm ET
Speakers: Claudie Beaulieu, Assistant Professor of Ocean Sciences, University of California, Santa Cruz and Rebecca Killick, Professor of Statistics, School of Mathematical Sciences, Lancaster University; Moderator: Emily Casleton, Statistical Sciences Group, Los Alamos National Laboratory (LANL)
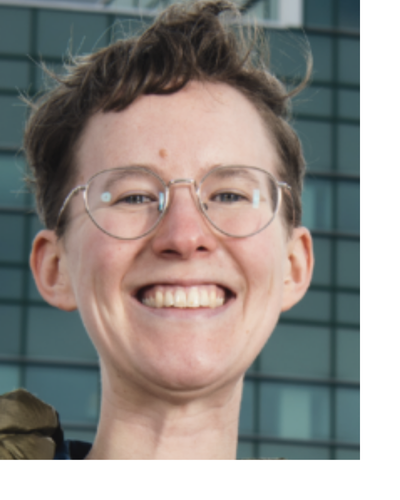
Astronomy & Cosmic Emulation
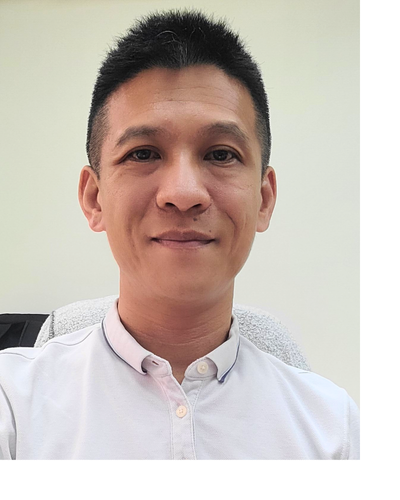
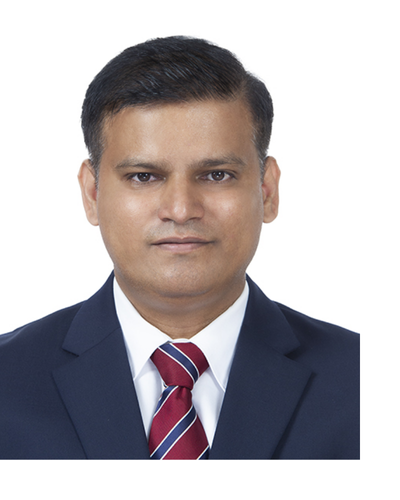