Quasi Stochastic Approximation with Applications to Gradient Free Optimization and Reinforcement Learning
Speaker
Sean Meyn, (University of Florida)
Abstract
Soon after the publication of Watkins’ Q-learning algorithm, it was recognized independently by Tsitsiklis and Jordan that the recursion could be analyzed within the framework of stochastic approximation (SA). The rich theory of SA provides simple convergence proofs, and also explains why many RL algorithms are so slow to converge.
This lecture surveys general theory of quasi stochastic approximation, in which random probing is replaced with deterministic signals such as sinusoids. The setting has great pedagogical value since it is relatively easy to obtain proofs of convergence, and bounds on rate of convergence. The practical implications are far more profound: it is easy to obtain 1/t rate of convergence in this deterministic setting, while the best rate of convergence is much slower when using SA. The talk will give a high level survey of the general theory, and how it can be applied to optimization and control.
References:
[1] S. Chen, A. Bernstein, A. Devraj, and S. Meyn. Stability and acceleration for quasi stochastic approximation. arXiv:2009.14431, 2020.
[2] Lecture notes for Theory of Reinforcement Learning Boot Camp https://simons.berkeley.edu/workshops/rl-2020-bc (draft manuscript: Control systems and reinforcement learning. To appear, Cambridge University Press)
Bio
Sean Meyn received the B.A. degree in mathematics from the University of California, Los Angeles (UCLA), in 1982 and the Ph.D. degree in electrical engineering from McGill University, Canada, in 1987 (with Prof. P. Caines, McGill University). He is now Professor and Robert C. Pittman Eminent Scholar Chair in the Department of Electrical and Computer Engineering at the University of Florida, the director of the Laboratory for Cognition & Control, and director of the Florida Institute for Sustainable Energy. His academic research interests include theory and applications of decision and control, stochastic processes, and optimization. He has received many awards for his research on these topics, and is a fellow of the IEEE.
He has held visiting positions at universities all over the world, including the Indian Institute of Science, Bangalore during 1997-1998 where he was a Fulbright Research Scholar. During his latest sabbatical during the 2006-2007 academic year he was a visiting professor at MIT and United Technologies Research Center (UTRC).
His award-winning 1993 monograph with Richard Tweedie, Markov Chains and Stochastic Stability, has been cited thousands of times in journals from a range of fields. The latest version is published in the Cambridge Mathematical Library.
For the past ten years his applied research has focused on engineering, markets, and policy in energy systems. He regularly engages in industry, government, and academic panels on these topics, and hosts an annual workshop at the University of Florida.
Event Type
- NISS Sponsored
Sponsor
Website
Location
Policy
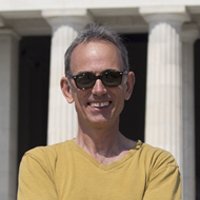