Overview
Generative language models like ChatGPT and others are rapidly changing the landscape of artificial intelligence. As these models become more prevalent, they raise numerous theoretical and practical questions about their use, limitations, and potential for bias. In this webinar, we address some of these questions and delve into strategies for mitigating bias in Large Language Models (LLMs) using the concept of multi-calibration. We also explore the evolution of neural networks beyond their traditional role as universal function approximators. These networks reveal their algorithmic prowess in modern generative AI models, challenging classical views of their capabilities. Moreover, we investigate the applications of clinical language models in healthcare predictions. These models can potentially revolutionize decision-making processes at the point of care, offering valuable insights for physicians and administrators. Join us for this webinar, where we unravel the complexities of AI, discuss innovative solutions, and explore its applications.
Speakers
Prof. Linjun Zhang, Rutgers
Prof. Song Mei, UC Berkeley
Lavender Jiang, PhD Student, NYU
Moderator
Dr. Nan Wu, Merck&Co.
Agenda
Prof, Linjun Zhang, Rutgers
Title: Mitigating Biases in LLMs: a General Framework of Fair Risk Control
Abstract: Recent studies have found that Large Language Models (LLMs) exhibit gender or racial disparities. In this talk, we will introduce a general framework of post-processing LLMs to reduce the bias. This framework is inspired by multi-calibration, a powerful and evolving concept originating in the field of algorithmic fairness. This general framework can also be applied to computer vision, uncertainty quantification, and transfer learning. In addition, if time permits, I will talk about the hallucination and creativity in LLMs.
Prof. Song Mei, UC Berkeley
Title: Revisiting Neural Network approximation theory in the age of generative AI
Abstract: Textbooks on deep learning theory primarily perceive neural networks as universal function approximators. While this classical viewpoint is fundamental, it inadequately explains the impressive capabilities of modern generative AI models such as language models and diffusion models. This talk puts forth a refined perspective: neural networks often serve as algorithm approximators, going beyond mere function approximation. I will explain how this refined perspective offers a deeper insight into the success of modern generative AI models.
Lavender Jiang, PhD student, NYU
Title: Health system-scale language models are all-purpose prediction engines
Abstract: Physicians make critical time-constrained decisions every day. Clinical predictive models can help physicians and administrators make decisions by forecasting clinical and operational events. Existing structured data-based clinical predictive models have limited use in everyday practice owing to complexity in data processing, as well as model development and deployment. Here we show that unstructured clinical notes from the electronic health record can enable the training of clinical language models, which can be used as all-purpose clinical predictive engines with low-resistance development and deployment. Our approach leverages recent advances in natural language processing to train a large language model for medical language (NYUTron) and subsequently fine-tune it across a wide range of clinical and operational predictive tasks. We evaluated our approach within our health system for five such tasks: 30-day all-cause readmission prediction, in-hospital mortality prediction, comorbidity index prediction, length of stay prediction, and insurance denial prediction. We show that NYUTron has an area under the curve (AUC) of 78.7–94.9%, with an improvement of 5.36–14.7% in the AUC compared with traditional models. We additionally demonstrate the benefits of pretraining with clinical text, the potential for increasing generalizability to different sites through fine-tuning and the full deployment of our system in a prospective, single-arm trial. These results show the potential for using clinical language models in medicine to read alongside physicians and provide guidance at the point of care.
About the Speakers
Prof, Linjun Zhang is an Assistant Professor in the Department of Statistics, at Rutgers University. He received my Ph.D. in Statistics at University of Pennsylvania in 2019 where he was advised by Professor T. Tony Cai. His current research interests include machine learning theory (especially deep learninig), high dimensional statistical inference, unsupervised learning and privacy-preserving data analysis.
Prof, Song Mei is an Assistant Professor in the Department of Statistics and the Department of Electrical Engineering and Computer Sciences at UC Berkeley. In June 2020, He received a Ph. D. from Stanford, where he was advised by Andrea Montanari. His research is motivated by data science and AI, and lies at the intersection of statistics, machine learning, information theory, and computer science. His research interests include theory of deep learning, theory of reinforcement learning, and high dimensional probability. His recent work focuses on the theoretical foundations for foundation models.
Lavender Yao Jiang is a PhD student at the NYU Center for Data Science. Prior to studying at NYU, Lavender graduated from Carnegie Mellon majoring in Electrical and Computer Engineering and Mathematical Sciences. She is interested in natural language processing and its application in healthcare. She is the lead researcher and principle engineer of NYUTron, one of the world’s largest and most sophisticated efforts to study large language models in healthcare. While at CMU, Lavender had the pleasure of working on signal processing, neuroscience, and robotics projects with Drs. José M.F. Moura, Pulkit Gover, and Howie Choset. In her free time, Lavender enjoys cooking, bouldering, and playing video games.
About the Moderator
Dr. Nan Wu is a senior scientist at Merck & Co., working on AI for precision medicine. She obtained her Ph.D. from the NYU Center for Data Science, where she worked on explainable AI in the context of multi-modal learning. She has built models to detect breast cancer and to predict the prognosis of COVID-19 patients, where diverse data sources, including medical images, EHR, and notes, have been explored. During her PhD, she also conducted research at Google Research and Amazon AWS AI Lab, working on foundation models for speech and text or image and text. Dr. Wu has published extensively in top conferences and journals in AI and medical domains. Her honors include a Google Ph.D. Fellow, Top 80 Global Young Chinese Women Scholars in AI (Baidu Academic, 2023), and Best Paper at an ICML workshop.
Event Type
- NISS Hosted
- NISS Sponsored
Host
Sponsor
Cost
Website
Location
Policy
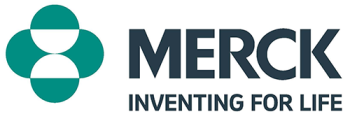