Speakers
Scott Lee (CDC)
"HaMLET: Enabling Quality Control at Scale for CDC’s Immigrant and Refugee Health Screening Program"
Luca Sartore (USDA-NASS/NISS)
"Predictive Cropland Data Layer: From Images to Statistics"
Kusuma Prabhakara (Census)
"U.S. Census Bureau Geography Division Satellite-Based Detection and Delineation of Built Environment Features"
Andy Ramlatchan (Department of Defense)
"Advancements in Statistical Learning and AI"
Moderator
Linda Young (USDA-NASS)
Agenda
Scott Lee
HaMLET: Enabling Quality Control at Scale for CDC’s Immigrant and Refugee Health Screening Program
Abstract: Immigrants and refugees seeking admission to the United States must first undergo an overseas medical exam, overseen by the US Centers for Disease Control and Prevention (CDC), during which all persons ≥15 years old receive a chest x-ray to look for signs of tuberculosis. Although individual screening sites often implement quality control (QC) programs to ensure radiographs are interpreted correctly, the CDC does not currently have a method for conducting similar QC reviews at scale. We obtained digitized chest radiographs collected as part of the overseas immigration medical exam. Using radiographs from applicants 15 years old and older, we trained deep learning models to perform three tasks: identifying abnormal radiographs; identifying abnormal radiographs suggestive of tuberculosis; and identifying the specific findings (e.g., cavities or infiltrates) in abnormal radiographs. We then evaluated the models on both internal and external testing datasets, focusing on two classes of performance metrics: individual-level metrics, like sensitivity and specificity, and sample-level metrics, like accuracy in predicting the prevalence of abnormal radiographs. A total of 152,012 images (one image per applicant; mean applicant age 39 years) were used for model training. On our internal test dataset, our models performed well both in identifying abnormalities suggestive of TB (area under the curve [AUC] of 0.97; 95% confidence interval [CI]: 0.95, 0.98) and in estimating sample-level counts of the same (-2% absolute percentage error; 95% CIC: -8%, 6%). On the external test datasets, our models performed similarly well in identifying both generic abnormalities (AUCs ranging from 0.89 to 0.92) and those suggestive of TB (AUCs from 0.94 to 0.99). This performance was consistent across metrics, including those based on thresholded class predictions, like sensitivity, specificity, and F1 score. Strong performance relative to high-quality radiological reference standards across a variety of datasets suggests our models may make reliable tools for supporting chest radiography QC activities at CDC.
Luca Sartore
Predictive Cropland Data Layer: From Images to Statistics
Abstract: Artificial Intelligence (AI) can be used to analyze aerial images and remotely sensed data, track farming decisions across a sequence of growing seasons, and forecast the crops to be planted during the upcoming growing season. Luca Sartore explores the application of AI methods to map the predicted crop types within the conterminous United States. Because these predictive maps are uncertain in nature, a map of the entropy associated with the predicted crops allows the final user to assess the utility of specific predictions. Finally, Luca will summarize how these maps aid with the automation and improvement of statistical methods at the United States Department of Agriculture's (USDA's) National Agricultural Statistics Service (NASS).
Andy Ramlatchan
Advancements in Statistical Learning and AI
As aerospace technologies advance, the demand for autonomous systems capable of complex decision-making continues to grow. This presentation delves into the evolution and utilization of statistical learning, machine learning (ML), and artificial intelligence (AI) in various autonomy-related applications. Focusing particularly on AI image analysis methods, we explore how these cutting-edge technologies are revolutionizing the way we perceive, analyze, and act upon visual data. Through real-world use cases and practical demonstrations, we showcase the transformative potential of statistical learning and AI algorithms in enhancing efficiency, safety, and reliability. Join us as we unravel the intricate synergy between advanced data-driven techniques and autonomy, paving the way for the future of intelligent systems.
Kusuma Prabhakara
U.S. Census Bureau Geography Division Satellite-Based Detection and Delineation of Built Environment Features
Abstract:The Geography Division of the Census Bureau has developed an automated change detection process using Artificial Intelligence and Machine Learning (AI/ML) to perform updates and maintenance to the Master Address File/Topologically Integrated Geographic Encoding and Referencing (MAF/TIGER) System. Using Sentinel-2 satellite imagery and Google Earth Engine, data scientists can apply large-scale cloud compute with open-source python scripts to multiple vintages of moderate resolution imagery to identify areas of new construction. Once these areas are identified, staff use machine learning to perform object extraction to capture the building footprint for new structures. These building footprints will enhance the accuracy of incoming addresses data, as well the existing address locations, and will serve as a reference for all spatial updates in the MAF/TIGER System. In addition, the use of automated change detection methods and AI/ML creates operational efficiencies for geographers by focusing attention on areas in which change has occurred and updates are needed and new geospatial data may need to be acquired.
About the Speakers
Kusuma Prabhakara, PhD, is a Geographer in the Geospatial Reference Data Branch (GRDB) within the Geography Division (GEO) at the U.S. Census Bureau. She is the imagery lead on the Automated Change Detection Core (ACDC) project which aims to utilize moderate- and high-resolution imagery to focus on areas of detected change and use machine learning (ML) algorithms to extract building footprints for use in our Intelligence Database (ID). Prior to joining the Census Bureau, Kusuma was a Graduate Research Assistant for the USDA’s Agricultural Research Service Hydrology and Remote Sensing Lab (ARS-HRSL) working on remote sensing of winter cover crops. This research culminated in a PhD from the Department of Geographical Sciences at the University of Maryland, College Park.
Scott Lee came to CDC in 2014 as an ORISE research participant in the National Center for HIV, Viral Hepatitis, STD, and TB Prevention’s Division of Tuberculosis Elimination, where he focused primarily on the statistical design of studies for improving TB case identification and diagnosis. In 2015, he moved to the Office of the Director in the Center for Surveillance, Epidemiology, and Laboratory Services, where he joined the Machine Intelligence and Data Science Team and worked on a wide variety of projects involving the application of machine learning to problems in public health surveillance, outbreak response, forecasting, and diagnostics. In 2023, he completed a four-month detail to the Coronavirus and Other Respiratory Viruses Division in the National Center for Immunization and Respiratory Diseases. While on detail, Scott served as the acting lead for the Biostatistics, Economics, and Model Unit in the Office of the Director and contributed to the development of methods for assessing interference between co-circulating respiratory viral pathogens in pediatric populations.
Luca Sartore is a Senior Research Associate for the National Institute of Statistical Science (NISS), working with the National Agricultural Statistical Service (NASS). He has been involved with the estimation and calibration of the US Census of Agriculture. He worked on modelling livestock, yield, and acreage for major agricultural commodities using various data sources, and he has also developed methodologies for assessing uncertainties. His contribution on the automation of analytical systems has focused on machine learning, artificial intelligence, and high-performance computing. He received his master in Statistics from the Ca’ Foscari University of Venice (Italy) and Ph.D. from the University of Padua (Italy). After his Ph.D., he joined the European Center of Living Technologies as a postdoc researching evolutionary algorithms in AI for one year in Venice (Italy). Since 2013, he has maintained several packages on the Comprehensive R-Archive Network (CRAN), one of which is currently used for production at NASS.
Andy Ramlatchan recently took a position with the US Department of Defense. Andy was formerly a systems researcher for the Engineering Integration Branch at NASA Langley Research Center, Andy's work involves the development and application of statistical learning, machine learning and artificial intelligence for a variety of autonomy related aerospace applications for spaceflight and aeronautics. Prior to this, he worked in cybersecurity strategy, development, and research for the United States government. I’m currently interested in developing high dimensional tensor completion methods for remote sensing systems for intelligent proactive systems security.
About the Moderator
Linda J. Young is Chief Mathematical Statistician and Director of Research and Development of USDA’s National Agricultural Statistics Service (NASS). She works with others within and outside of NASS to continually improve the methodology underpinning the Agency’s collection and dissemination of data on every facet of U.S. agriculture. Her recent research has focused on the use of open-source data, capture-recapture methodology, and integrating survey and non-survey data to produce estimates. Linda served on the faculties of three U.S. land grant universities: Oklahoma State University, University of Nebraska, and the University of Florida before joining NASS. Linda has authored or co-authored four books and more than 100 publications in over 50 different journals, constituting a mixture of statistics and subject-matter journals. She is an emphasis editor for the Statistical Journal of the IAOS and past editor of the Journal of Agricultural, Biological, and Environmental Statistics. She has served in a broad range of offices within the professional statistical societies, including President of the Eastern North American Region of the International Biometric Society, Vice-President of the American Statistical Association, Treasurer of the International Biometric Society, Chair of Section U (Statistics) of the American Association for the Advancement of Science (AAAS), Chair of the Committee of Presidents of Statistical Societies and Council Member of the International Statistical Institute (ISI). Linda is a fellow of the American Statistical Association (ASA), a fellow of AAAS, and an elected member of the ISI.
About the NISS/FCSM AI in Federal Government Series
The National Institute of Statistical Sciences (NISS) and the Federal Committee on Statistical Methodology (FCSM) are collaborating on a series of webinars on Artificial Intelligence (AI). The initial webinar took place on October 31, 2023 on AI in Federal Government: Uses, Potential Applications, and Issues. This series aims to benefit federal practitioners and managers by providing behind-the-scenes information on uses of AI in federal agencies and from insights on how agencies meet organizational, managerial, and ethical challenges in harnessing the power of AI. Participation by researchers and managers in the webinars can help streamline current efforts to adopt AI and inspire new endeavors. The NISS/FCSM webinar series creates unique opportunities not easily available through other forums or venues. Thank you to the American Statistical Association (ASA), NORC at the Univesity of Chicago, & RTI International for sponsoring of the series.
Event Type
- NISS Hosted
Host
Sponsor
Website
Location
Policy
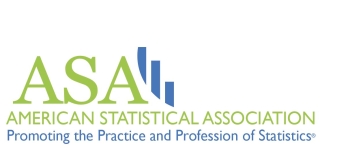
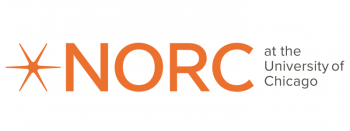
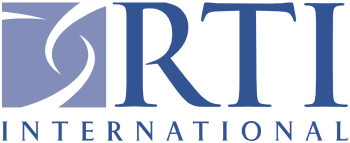