Please note that this event has already taken place. You may view the news story and recording here: https://www.niss.org/news/niss-launches-collaboratory-and-inaugurates-ai...
Speaker:
Bin Yu, Statistics, EECS, Center for Comp. Bio. And Simons Institute
Abstract:
The rapid advancement of AI relies heavily on the foundation of data science, yet its education significantly lags its demand in practice. The upcoming book 'Veridical Data Science: The Practice of Responsible Data Analysis and Decision Making' (Yu and Barter, MIT Press, 2024; free online at www.vdsbook.com) tackles this gap by promoting Predictability, Computability, and Stability (PCS) as core principles for trustworthy data insights. PCS for veridical data science (VDS) has been developed in the process of solving scientific data science problems. It thoroughly integrates these principles into the Data Science Life Cycle (DSLC), from problem formulation to data cleansing and to result communication, fostering a new standard for responsible data analysis. This talk explores PCS' motivations, and compare the VDS book appraoch with traditional ones. Then I will describe two PCS projects on prostate cancer detection and discovery of epistastic genetic drivers for a heart disease. I will end with on-going work for PCS uncertainty quantificaiton in regression and its comparison with conformal prediction, PCS software pacakages (v-flow, simChe), and MEIRTS guidelines for data-inspired simulations.
About the Speaker:
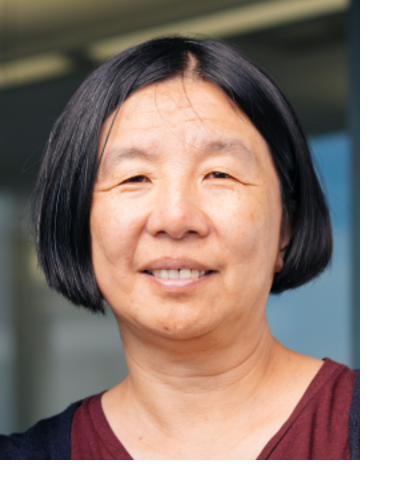
About the Moderator:
Nancy McMillan currently serves as Data Science Research Leader within Battelle’s Health Research & Analytics Business Line. For a diverse set of federal government clients, she currently leads development of a large language model (LLM) based biocuration acceleration pipeline and user tool, development of pipelines, analytics, and visualizations of electronic initial case reporting data, and development of analytical methods for achieving abbreviated new drug application (ANDA) approval for an agile drug manufacturing technology. Nancy has a long history of collaborative work across Battelle bringing statistics and machine learning to Battelle’s deep capability in biology, chemistry, and material science. As a researcher and Project Management Professional, Nancy has worked and published on environmental exposure and risk assessment; transportation safety benefits; quantitative risk assessment related to chemical, biological, radiological and nuclear (CBRN) terrorism; bio surveillance; and bioinformatics. She managed the Health Analytics Division from 2017-2023, a team of approximately 100 data scientists that supports Battelle’s contract research business. Nancy is a member of the Board of Trustees for the National Institute of Statistical Sciences (NISS), the Chair of NISS’s Affiliates Committee, and a member of the Organ Procurement and Transplantation Network’s Data Advisory Committee.
About AI, Data Science, and Statistics in Practice
The NISS AI, Data Science, and Statistics in Practice is a monthly event series part of the NISS Collaboratory (CoLab) will bring together leading experts from industry and academia to discuss the latest advances and practical applications in AI, data science, and statistics. Each session will feature a keynote presentation on cutting-edge topics, where attendees can engage with speakers on the challenges and opportunities in applying these technologies in real-world scenarios. This series is intended for professionals, researchers, and students interested in the intersection of AI, data science, and statistics, offering insights into how these fields are shaping various industries. The series is designed to provide participants with exposure to and understanding of how modern data analytic methods are being applied in real-world scenarios across various industries, offering both theoretical insights, practical examples, and discussion of issues.
Featured Topics:
- Veridical Data Science - Speaker: Bin Yu, October 15,2024
- Random Forests: Why they Work and Why that’s a Problem - Speaker: Lucas Mentch, November 19, 2024
- Causal AI in Finance and Technology Industries - Speakers: Victor Lo, and Victor Chen, January 24, 2025
- Large Language Models: Transforming AI Architectures and Operational Paradigms - Speaker: Frank Wei, February 18, 2025
- Experimental Design and Causal Inference
- Statistics and Experimentation Needs in Industry
- Generative AI for Use in Industry
- Uncertainty Quantification for Random Forests
- Deep Learning Methods for Closed-Loop Neuromodulation
- Machine Learning for Airborne Biological Hazard Detection
- Causal Inference in Marketing Analytics
- Practical Return on AI Investment
Event Type
- NISS Hosted
Website
Location
Policy
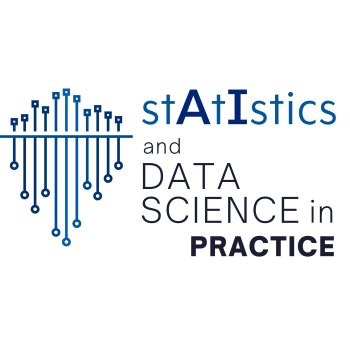