Please Note: This event has already taken place, you can view the News Story and Recording here: Unraveling Causality: NISS Webinar Explores AI and Causal Inference in Finance and Tech | National Institute of Statistical Sciences
Overview
This webinar will explore the growing role of causal AI in uncovering cause-and-effect relationships within complex systems. The session will highlight how causal AI differs from traditional predictive models, emphasizing its potential to improve decision-making across various domains. Attendees will gain insights into techniques for measuring the impact of interventions and understanding causal mechanisms. Broader examples will illustrate its application in optimizing strategies and enhancing outcomes. Key challenges, such as data reliability and model validation, will also be explored. The webinar will conclude with practical guidance on leveraging causal AI in dynamic and high-impact settings.
Speakers
Victor Lo, Senior Vice President of Data Science and Artificial Intelligence, Workplace Investing at Fidelity Investments
Victor Zitian Chen, PhD, Head of Science Excellence, PXT Central Science at Amazon
Moderator
Nancy McMillan, Data Science Research Leader, Health Research & Analytics Business Line at Battelle
Abstract
We will first discuss why we should care about causal inference and then provide an overview of three major schools of thought and their key approaches from the disciplines of Statistics, Epidemiology, Computer Science, and Economics/Econometrics. The techniques developed in these fields have been applied to a wide range of fields including medical sciences, economics, political science, education, and business analytics. Their successes in the real world have led to winning a Turing Award (2011) and Nobel Prizes in Economics (2019, 2021). We will then dive deep to explore the intersection between causal inference and AI in solving business problems. Extension to uplift modeling will also be covered in this seminar. Various real-life applications based on different approaches will be used for illustration.
Event Disclaimer
The views and opinions expressed by the speakers during this event are their own and do not necessarily reflect the views, positions, or policies of their employers, affiliated organizations, or any other entity. The speakers are participating in a personal capacity, and their statements should not be attributed to their respective companies or institutions.
About the Speakers
Victor S.Y. Lo is a seasoned Big Data, Marketing, Risk, and Finance leader & innovator with over two decades of extensive consulting and corporate experience employing data-driven solutions in a wide variety of business areas, including Customer Relationship Management, Market Research, Advertising Strategy, Risk Management, Financial Econometrics, Insurance, Product Development, Transportation, Healthcare, and Human Resources. He is actively engaged with Big Data Analytics, causal inference, and is a pioneer of Uplift/True-lift modeling, a key subfield of data science that has been applied to areas as marketing, political election, and medicine. Victor has served as the manager of quantitative teams in multiple organizations. He is currently Senior Vice President of Data Science and Artificial Intelligence in Workplace Solutions at Fidelity Investments. Previously he managed advanced analytics teams in Personal Investing, Corporate Treasury, Managerial Finance, and Healthcare and Total Well-being at Fidelity Investments. Prior to Fidelity, he was VP and Manager of Modeling and Analysis at FleetBoston Financial (now part of Bank of America), and Senior Associate at Mercer Management Consulting (now Oliver Wyman). For academic & industry services, Victor has been a visiting research fellow and corporate executive-in-residence at Bentley University. He has also been serving on the steering committee of the Boston Chapter of the Institute for Operations Research and the Management Sciences (INFORMS) and on the editorial board for two academic journals. He is a frequently invited speaker at several conferences, bridging the gap between industry and academia. Victor has a master’s degree in Operational Research from Lancaster University and a PhD in Statistics from the University of Hong Kong, and was a Postdoctoral Fellow in Management Science at the University of British Columbia. He is a co-editor of a graduate level econometrics book, published numerous articles in Data Mining, Marketing, Statistics, and Management Science literature, and is finishing a graduate level text book on causal inference for business.
Victor Zitian Chen, PhD, is Head of Science Excellence at Amazon People eXperience and Technology (PXT) Central Science. There, he is leading an interdisciplinary group of scholars, scientists, and product/program leaders to raise the bar of research into decisions and products, centralize the institutional knowledge, and build an ecosystem of science-related tools. Before Amazon, Victor was the director/VP of Data analytics and insights, experimental design and causal inference at Fidelity Investments. At Fidelity, he led the development and deployment of the first enterprise-wise platform for Causal and eXperiemntal Analytics (CAX). Before industry, Victor was a tenured professor in strategy and international business, with posts at Copenhagen Business School and UNC Charlotte. As a scholar-turned tech entrepreneur, he has built two start-ups and holds two pending patents. He was recently listed on the Business North Carolina Power List 2024 and named a CDO Magazine finalist for 2024 Top 40 under 40 Data Leaders among other recognitions.
About the Moderator
Nancy McMillan currently serves as Data Science Research Leader within Battelle’s Health Research & Analytics Business Line. For a diverse set of federal government clients, she currently leads development of a large language model (LLM) based biocuration acceleration pipeline and user tool, development of pipelines, analytics, and visualizations of electronic initial case reporting data, and development of analytical methods for achieving abbreviated new drug application (ANDA) approval for an agile drug manufacturing technology. Nancy has a long history of collaborative work across Battelle bringing statistics and machine learning to Battelle’s deep capability in biology, chemistry, and material science. As a researcher and Project Management Professional, Nancy has worked and published on environmental exposure and risk assessment; transportation safety benefits; quantitative risk assessment related to chemical, biological, radiological and nuclear (CBRN) terrorism; bio surveillance; and bioinformatics. She managed the Health Analytics Division from 2017-2023, a team of approximately 100 data scientists that supports Battelle’s contract research business. Nancy is a member of the Board of Trustees for the National Institute of Statistical Sciences (NISS), the Chair of NISS’s Affiliates Committee, and a member of the Organ Procurement and Transplantation Network’s Data Advisory Committee.
About AI, Statistics and Data Science in Practice
The NISS AI, Statistics and Data Science in Practice is a monthly event series will bring together leading experts from industry and academia to discuss the latest advances and practical applications in AI, data science, and statistics. Each session will feature a keynote presentation on cutting-edge topics, where attendees can engage with speakers on the challenges and opportunities in applying these technologies in real-world scenarios. This series is intended for professionals, researchers, and students interested in the intersection of AI, data science, and statistics, offering insights into how these fields are shaping various industries. The series is designed to provide participants with exposure to and understanding of how modern data analytic methods are being applied in real-world scenarios across various industries, offering both theoretical insights, practical examples, and discussion of issues.
Featured Topics:
- Veridical Data Science - Speaker: Bin Yu, October 15,2024
- Random Forests: Why they Work and Why that’s a Problem - Speaker: Lucas Mentch, November 19, 2024
- Causal AI in Finance in Business Practices - Speakers: Victor Lo, and Victor Chen, January 24, 2025
- Large Language Models: Transforming AI Architectures and Operational Paradigms - Speaker: Frank Wei, February 18, 2025
- Machine Learning for Airborne Biological Hazard Detection - Speaker: Jared Schuetter, March 11, 2025
- Experimental Design and Causal Inference
- Statistics and Experimentation Needs in Industry
- Generative AI for Use in Industry
- Uncertainty Quantification for Random Forests
- Deep Learning Methods for Closed-Loop Neuromodulation
- Causal Inference in Marketing Analytics
- Practical Return on AI Investment
Event Type
- NISS Hosted
Host
Website
Location
Policy
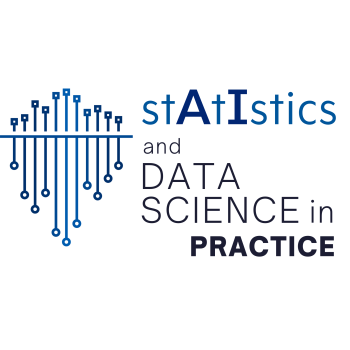