[Please Note: The Essential Data Science for Business: Domain Knowledge and Application Areas has already occurred.
Go to the News Story for this event to read about what happened., or...
Missed this Event? To gain access to the recording of this event along with links to supporting files and information, complete the Registration Option for "Post Session Access" on the right hand side of this webpage.]
The tutorials in this NISS series involve the Top 10 analytics approaches of the key topics that are used in business today! Students and faculty, these are perhaps the top ten most important and practical topics that may not be covered in your program of study. (Review the Overview Presentation about all 10 Sessions).
Domain Knowledge and Application Areas
This tutorial features a review of three different case studies found in business today. Below are short descriptions presentations
Artificial Intelligence and Machine Learning Applications in Banking
Jie Chen – Wells Fargo
Artificial intelligence and machine learning have been rapidly adopted across financial institutions to address new challenges and improve business decisions as well as customer experiences. This presentation will describe various machine learning techniques and their applications in banking: credit risk forecasting, trading and market risk models, and finance crime models. New merging challenges and applications such as chat bots, complaint analysis, and customer assistance and their solutions will also be introduced. Real case studies in banking will be used to illustrate the results.
Surveys and Big Data for Estimating Brand Lift
Tim Hesterberg – Google
Google Brand Lift Surveys estimates the effect of display advertising using surveys. Challenges include imperfect A/B experiments, response and solicitation bias, discrepancy between intended and actual treatment, comparing treatment group users who took an action with control users who might have acted, and estimation for different slices of the population. We approach these issues using a combination of individual-study analysis and meta-analysis across thousands of studies. This work involves a combination of small and large data - survey responses and logs data, respectively. There are a number of interesting and even surprising methodological twists. We use regression to handle imperfect A/B experiments and response and solicitation biases; we find regression to be more stable than propensity methods. We use a particular form of regularization that combines advantages of L1 regularization (better predictions) and L2 (smoothness). We use a variety of slicing methods, that estimate either incremental or non-incremental effects of covariates like age and gender that may be correlated. We bootstrap to obtain standard errors. In contrast to many regression settings, where one may either resample observations or fix X and resample Y, here only resampling observations is appropriate. (Attendees will be interested in reviewing this link to a paper that is very similar to the topics that Tim will be covering.)
Forecast Generation and Evaluation for Datacenter Capacity Planning
Juan Li – Google
Google owns and operates data centers all over the world, helping to keep the internet humming 24/7. We are a group of data scientists that focus on modeling capacity requirements for Google’s compute and storage resources. We use statistical models to learn organic demand patterns from historical data to balance stockout risk and efficiency of our data centers. Planning virtual resources has interesting challenges and opportunities. For example, some of our data can literally be stored anywhere in the world, while some of the data has specific location requirements. Hence, we need to forecast capacity requirements at various levels of granularities.
In this tutorial, I will illustrate how we use hierarchical time series range forecasts to tackle capacity planning challenges at Google. The tutorial will start with reviewing why range forecast is important for capacity planning, then followed by hierarchical time series range forecasts. The last section will focus on metrics evaluation.
Instructors
Jie Chen (Wells Fargo),
Tim Hesterberg (Google) and
Juan Li (Google)
Series Goals
NISS is interested in sharing knowledge. To this end, these tutorials have been geared to provide practical information that you can use tomorrow. Examples, projects and code sharing are a part of these sessions wherever possible.
Series Prerequisites
Participants require a working knowledge of probability distributions, statistical inference, statistical modeling and time series analysis as a prerequisite. Students who do not have this foundation or have not reviewed this material within the past couple of years will struggle with the concepts and methods that build on this foundation. Please Note: Each tutorial is presented as a stand-alone tutorial, in other words, you need not to have attended earlier sessions in order to attend later sessions.
Registration
Select a registration/payment option above the 'Register for this Event' button ($35 for this Data Science Essentials tutorial session, $250 for all 10 Essential Data Science for Business tutorial sessions.
Can't attend this session or any of the previous sessions? Post Session Access to tutorial materials and recording can be obtained for $35 after the event is over. Purchasing all 10 Sessions also will provide you access to all previous session recordings and materials.) NISS Affiliates, (https://www.niss.org/affiliates-list), please send an email to officeadmin@niss.org.). Notifications: You will recieve an email that comes immediately to let you know you paid. Links to the event will come via email the day before and one hour prior to the actual session.
Agenda
About the Instructors
Jie Chen is Managing Director in the Advanced Technologies for Modeling (AToM) Group of Corporate Model Risk at Wells Fargo. She is leading the Statistics and Machine Learning team, focusing on development of cutting-edge models, algorithms, and a computing platform to advance the Bank’s practice in the areas of credit, operational, and market risk management. She has over ten year experience on machine learning, artificial intelligence and advanced statistics in the banking industry. Jie holds a Ph.D. in Statistics from the Stewart School of Industrial and Systems Engineering at the Georgia Institute of Technology.
Tim Hesterberg is a Senior Data Scientist at Google. He previously worked at Insightful, Franklin & Marshall College, and Pacific Gas & Electric Co. He received his Ph.D. in Statistics from Stanford University, under Brad Efron, and is a Fellow of the American Statistical Association. He is author of the "Resample" package for R, Chihara and Hesterberg "Mathematical Statistics with Resampling and R" (2018), and "What Teachers Should Know about the Bootstrap: Resampling in the Undergraduate Statistics Curriculum", The American Statistician 2015.
Juan Li is a Senior Data Scientist at Google. She leads a data science team that develops resource planning models in the Technical Infrastructure division. She previously worked at Xerox Innovation Group as a research scientist. She received her Ph.D. in Operations Research from Cornell University with a focus on supply chain application, under Prof. John Muckstadt.
Event Type
- Affiliate Award Fund Eligible
- NISS Hosted
- NISS Sponsored
Location
Policy
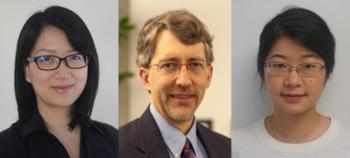