In 2017 the ASA published the statement, "The ASA's Statement on p-Values: Context, Process, and Purpose". Since that time, many statisticians have been thinking and writing about alternatives to the traditional p-value.
This work culminated in the publication of a special edition of The American Statistician. The title of this special issue is "Statistical Inference in the 21st Century: A World Beyond p < 0.05" that featured 43 papers on alternatives to the traditional use of p-values.
On May 23, 2019 NISS hosted a webinar discussing the major ideas covered in some of these papers. Recordings and slides for May webinar are available on the NISS website.
Following the very successful May webinar, NISS will take the conversations on p-values a step further by inviting three authors who published in the special TAS issue to share insights about their specific ideas on November 19, 2019 from 12 - 2 pm (ET). The three authors are Jim Berger, Sander Greenland, and Robert Matthews who will share personal insights into their own alternative approaches. The webinar will be moderated by Dan Jeske from UC Riverside and editor of The American Statistician.
The webinar will use Zoom and is free to the public. We invite you to register for this webinar using the registration link.
Agenda
Webinar Outline
12:00 – 12:05 Dan Jeske, Opening remarks and logistics
12:05 – 12:35 Jim Berger, Three recommendations for improving the use of p-values
12:35 – 13:05 Sander Greenland, Integrating causality, frequency, and Bayes using P-values and S-values
13:05 – 13:35 Robert Matthews, The analysis of credibility as a means of moving the scientific community beyond NHST
13:35 – 14:00 Dan Jeske and the presenters, Q&A and discussion
Abstracts
Jim Berger
"Three Recommendations for Improving the Use of p-Values"
Researchers commonly use p-values to answer the question: How strongly does the evidence favor the alternative hypothesis relative to the null hypothesis? P-values themselves do not directly answer this question and are often misinterpreted in ways that lead to overstating the evidence against the null hypothesis. Even in the post p < 0.05 era, however, it is quite possible that p-values will continue to be widely reported and used to assess the strength of evidence. If so, the potential for misinterpretation will persist. In this paper, we recommend three practices that would help researchers more accurately interpret p-values. Each of the three recommended practices involves interpreting p-values in light of the largest odds in favor of the alternative hypothesis relative to the null hypothesis that is consistent with the observed data.
Sander Greenland
"Integrating causality, frequency, and Bayes using P-values and S-values"
There are huge gaps in current statistical theory and education which contribute to its opacity to researchers and impede meeting user needs to summarize and interpret data in valid, relevant, transparent ways. By focusing on probability theory, statistics limits itself to extraction of digitally coded information. In research on poorly understood complex phenomena, however, its assumptions and conclusions depend on highly biased observer perceptions and judgments. Statistics centered on probability theory thus needs to be replaced by an integration of causal modeling, data description, information extraction, and cognitive science, with probability entering as but one of the mathematical tools for delineating these elements.
To transparently connect data to models and context, this integration focuses on how data features (“statistics”) causally depend on both the target features (“population parameters”) and the data-generator features (“design parameters”). These features are supposed to be captured by a statistical model, which should be accompanied by contextually plausible mechanical (physical, causal) explanations for each model assumption. In this fashion, causality theory is essential for all studies, whether comparative or purely descriptive (e.g., surveys and polling, in which the target is a noncausal population feature).
As long recognized by pragmatic Bayesians, calibration of models against data is essential. For this task, P-values re-emerge as basic calibration tools. Nonetheless, they need to be reinterpreted unconditionally as providing relations between data sets and models, not as model conditioned tests of specific hypotheses. To aid that task, a P-value p can be transformed into an S-value s = log(1/p) = −log(p), a “standardized” measure of distance from the model to the data scaled in information units. Finally, assumptions and interpretations (inferences, conclusions) need to be evaluated against a list of the biases (both methodologic and cognitive) that often dominate reports, especially when vested interests are operating.
Robert Matthews
"The Analysis of Credibility as a means of moving the scientific community beyond NHST"
The statistical community has long warned researchers that their go-to method of turning data into insight - Null Hypothesis Significance Testing (NHST) – is unfit for purpose. To date, these warnings have led to no systemic change in inferential practices. One plausible explanation is that abandoning NHST fails the pragmatic “cost-benefit analysis” in which the benefits of acquiring expertise in alternative inferential methods are weighed against the risk of challenge from, among others, editors and referees of leading journals.
This suggests that efforts to move the scientific community beyond NHST should be evolutionary rather than revolutionary. In this spirit, I outline the theory and practice of the Analysis of Credibility (AnCred), a Bayesian methodology which extracts additional inferential insight from standard data summaries. Drawing on real-life examples, I argue that AnCred is a simple, intuitive and transparent means of “adding value” to both significant and non-significant findings. As such, it also reduces the risk of making standard inferential errors, including perhaps the most egregious: that p = 0.05 is the hard border between genuine and “null” findings.
About the Authors
Jim Berger is the Arts and Sciences Professor of Statistics at Duke University. His current research interests include Bayesian model uncertainty and uncertainty quantification for complex computer models. Berger was president of the Institute of Mathematical Statistics from 1995-1996 and of the International Society for Bayesian Analysis during 2004. He was the founding director of the Statistical and Applied Mathematical Sciences Institute, serving from 2002-2010. He was co-editor of the Annals of Statistics from 1998-2000 and was a founding editor of the Journal on Uncertainty Quantification from 2012-2015. Berger received the COPSS `President's Award’ in 1985, was the Fisher Lecturer in 2001, the Wald Lecturer of the IMS in 2007, and received the Wilks Award from the ASA in 2015. He was elected as a foreign member of the Spanish Real Academia de Ciencias in 2002, elected to the USA National Academy of Sciences in 2003, was awarded an honorary Doctor of Science degree from Purdue University in 2004, and became an Honorary Professor at East China Normal University in 2011.
Sander Greenland is Emeritus Professor of Epidemiology and Statistics at the University of California, Los Angeles. A Fellow of the American Statistical Association and the Royal Statistical Society, he is a leading contributor to epidemiologic methodology, with a focus on delineating and preventing the misuse of statistical methods in observational studies. He has published over 400 articles and book chapters in epidemiology, statistics, and medicine, and given over 250 invited lectures, seminars and courses worldwide in epidemiologic and statistical methodology.
Robert Matthews is Visiting Professor in the Department of Mathematics at Aston University, Birmingham. A graduate in physics from Oxford University, he is a Fellow of the Royal Statistical Society and a editorial board member for Significance. His publications on inference have dealt with issues ranging from barriers to the use of Bayesian methods in clinical research to the viability of earthquake forecasting.
Event Type
- NISS Hosted
Host
Cost
Website
Location
Policy
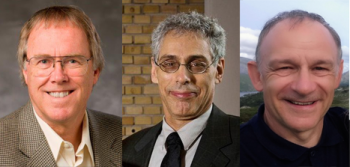