The COPSS-NISS Leadership Webinar: Leadership in Trustworthy AI brings together leading experts to discuss the critical role of leadership in developing and deploying AI systems that are ethical, transparent, and reliable. As AI continues to transform industries and decision-making processes, ensuring trustworthiness in AI-driven solutions is more important than ever. This webinar will explore key challenges and best practices in responsible AI leadership, covering topics such as fairness, accountability, interpretability, and risk management. Attendees will gain valuable insights from experienced leaders at the forefront of AI research and application, making this an essential event for professionals, researchers, and policymakers navigating the evolving AI landscape.
Panelists
David Donoho, Anne T. and Robert M. Bass Professor of Humanities and Sciences Professor of Statistics, Stanford University
Michael I. Jordan, Pehong Chen Distinguished Professor Emeritus, Department of EECS & Department of Statistics, University of California, Berkeley
Moderator
Tracy Ke, Associate Professor in the Department of Statistics at Harvard University
About the Panelists
David Donoho has studied the exploitation of sparse signals in signal recovery, including for denoising, superresolution, and solution of underdetermined equations. His research with collaborators showed that ell-1 penalization was an effective and even optimal way to exploit sparsity of the object to be recovered. He coined the notion of compressed sensing which has impacted many scientific and technical fields, including magnetic resonance imaging in medicine, where it has been implemented in FDA-approved medical imaging protocols and is already used in millions of actual patient MRIs. In recent years David and his postdocs and students have been studying large-scale covariance matrix estimation, large-scale matrix denoising, detection of rare and weak signals among many pure noise non-signals, compressed sensing and related scientific imaging problems, and most recently, empirical deep learning. See Academic Profile
Michael I. Jordan is the Pehong Chen Distinguished Professor in the Department of Electrical Engineering and Computer Science and the Department of Statistics at the University of California, Berkeley. He received his Masters in Mathematics from Arizona State University, and earned his PhD in Cognitive Science in 1985 from the University of California, San Diego. He was a professor at MIT from 1988 to 1998. His research interests bridge the computational, statistical, cognitive, biological and social sciences. Prof. Jordan is a member of the National Academy of Sciences, a member of the National Academy of Engineering, a member of the American Academy of Arts and Sciences, and a Foreign Member of the Royal Society. He is a Fellow of the American Association for the Advancement of Science. He was the inaugural winner of the World Laureates Association (WLA) Prize in 2022. He received the Ulf Grenander Prize from the American Mathematical Society in 2021, the IEEE John von Neumann Medal in 2020, the IJCAI Research Excellence Award in 2016, the David E. Rumelhart Prize in 2015, and the ACM/AAAI Allen Newell Award in 2009. He gave the Inaugural IMS Grace Wahba Lecture in 2022, the IMS Neyman Lecture in 2011, and an IMS Medallion Lecture in 2004. He was a Plenary Lecturer at the International Congress of Mathematicians in 2018. In 2016, Prof. Jordan was named the "most influential computer scientist" worldwide in an article in Science, based on rankings from the Semantic Scholar search engine. See Academic Profile
About the Moderator
Tracy Ke is an Associate Professor in the Department of Statistics at Harvard University. Her research focuses on high-dimensional statistics, statistical machine learning, network analysis, and large-scale inference. She has made significant contributions to developing methodologies for sparse PCA, community detection, and inference for high-dimensional models. Dr. Ke received her Ph.D. in Statistics from Princeton University and has since been recognized for her impactful work in statistical theory and applications. Her research has been published in top statistical journals, and she actively collaborates across disciplines to advance statistical methodologies for complex data structures. At Harvard, she teaches courses in statistical theory and methods while mentoring students and postdoctoral researchers. Her work continues to shape modern statistical research, particularly in areas involving large-scale and high-dimensional data problems. For more details on her research and publications, visit her website.
About the COPSS-NISS Leadership Webinar Series
COPSS (Committee of the Presidents of Statistical Societies) and NISS have come together to organize and host a new webinar series focusing on leadership in statistics and data science. Plan to attend these webinars every month during the academic year! Visit the COPSS-NISS Leadership Series Page for previous webinars.
The COPSS-NISS Leadership Webinar Series is co-organized by the Committee of the Presidents of Statistical Societies (COPSS) Emerging Leaders in Statistics and the National Institute of Statistical Sciences (NISS). The purpose of the webinar series is to promote leadership skills for members of the statistical societies at any stage in their careers. The series features conversations with leaders throughout the discipline, including leaders from major academic and government institutions, and companies. Invited speakers share their leadership stories and answer questions about their experiences. Each webinar is moderated by a member of the COPSS Emerging Leaders in Statistics program.
Access the Full COPSS-NISS Leadership Webinar Series YouTube Playlist | COPSS-NISS Leadership Webinar Series: https://www.youtube.com/playlist?list=PLoRtupvDJTjvFukMcO6NfDr0GvxDsIj81
Event Type
- NISS Hosted
- NISS Sponsored
Website
Location
Policy

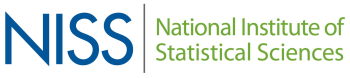